Research [in] Brief: Two COVID-19 Modeling Approaches
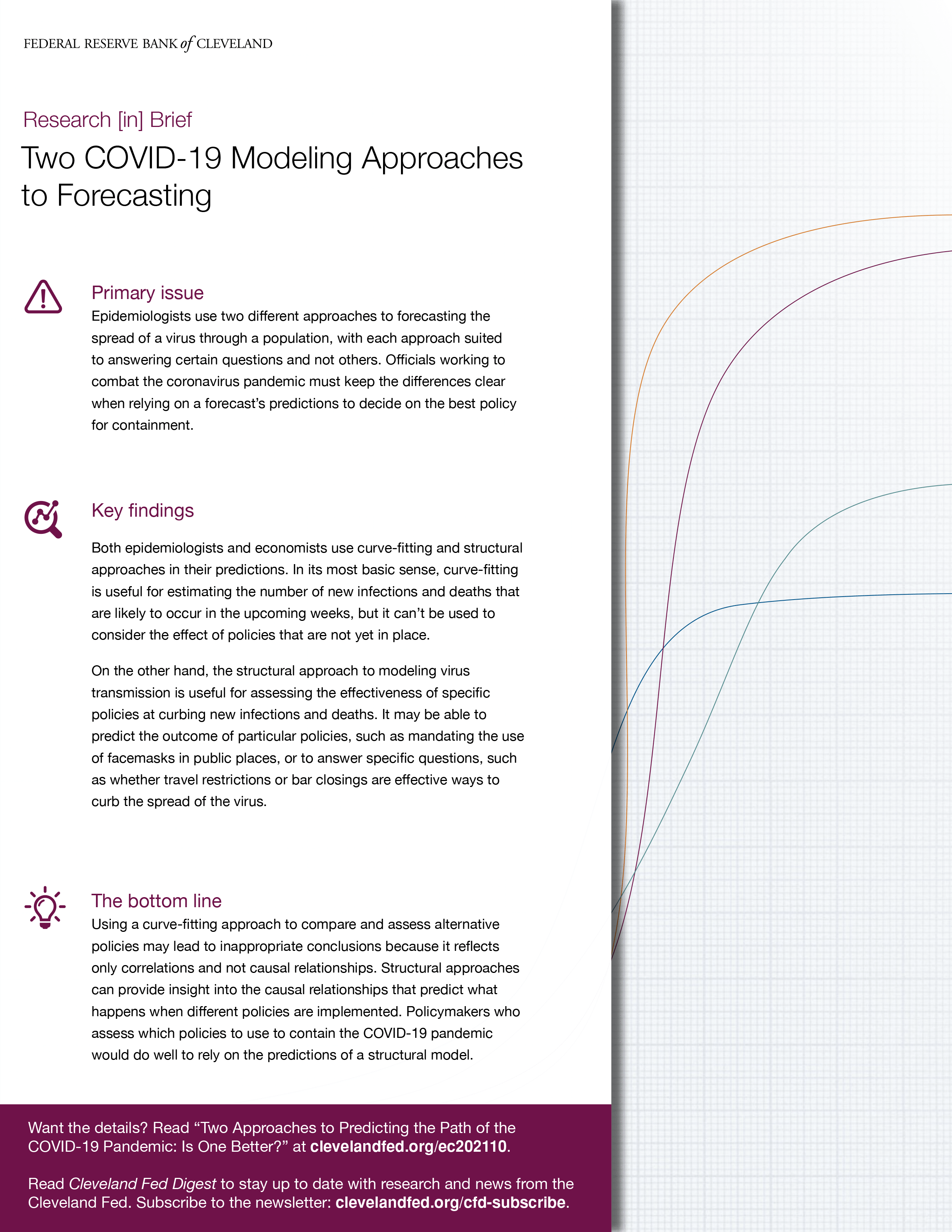
Primary issue
Epidemiologists use two different approaches to forecasting the spread of a virus through a population, with each approach suited to answering certain questions and not others. Officials working to combat the coronavirus pandemic must keep the differences clear when relying on a forecast’s predictions to decide on the best policy for containment.
Key findings
Both epidemiologists and economists use curve-fitting and structural approaches in their predictions. In its most basic sense, curve-fitting is useful for estimating the number of new infections and deaths that are likely to occur in the upcoming weeks, but it can’t be used to consider the effect of policies that are not yet in place.
On the other hand, the structural approach to modeling virus transmission is useful for assessing the effectiveness of specific policies at curbing new infections and deaths. It may be able to predict the outcome of particular policies, such as mandating the use of facemasks in public places, or to answer specific questions, such as whether travel restrictions or bar closings are effective ways to curb the spread of the virus.
The bottom line
Using a curve-fitting approach to compare and assess alternative policies may lead to inappropriate conclusions because it reflects only correlations and not causal relationships. Structural approaches can provide insight into the causal relationships that predict what happens when different policies are implemented. Policymakers who assess which policies to use to contain the COVID-19 pandemic would do well to rely on the predictions of a structural model.
Want to find out more? Read “Two Approaches to Predicting the Path of the COVID-19 Pandemic: Is One Better?” at clevelandfed.org/ec202110.
- Share