The CPI–PCEPI Inflation Differential: Causes and Prospects
The views authors express in Economic Commentary are theirs and not necessarily those of the Federal Reserve Bank of Cleveland or the Board of Governors of the Federal Reserve System. The series editor is Tasia Hane. This paper and its data are subject to revision; please visit clevelandfed.org for updates.
There are two official measures of inflation in consumer prices in the United States, one constructed using the consumer price index (CPI), produced by the Bureau of Labor Statistics (BLS), and the other constructed using the personal consumption expenditures price index (PCEPI), produced by the Bureau of Economic Analysis (BEA). The CPI is used more widely, by the federal government (for example, to index Social Security payments and to move income tax brackets), by businesses and state governments (for example, to index commercial real estate leases and to index the minimum wage in some states), and by financial markets (for example, for Treasury Inflation Protected Securities (TIPS) and inflation-linked derivatives). However, the Federal Open Market Committee (FOMC) states its official inflation target in terms of the PCEPI.1
Inflation rates measured by the CPI and PCEPI are generally similar; both track price changes in a basket of consumption goods and services, and the PCEPI makes use of much of the data from the CPI. But the indexes and their associated inflation rates are not identical. On average, since December 1978, 12-month CPI inflation has run 0.30 percentage points (ppts) above 12-month PCEPI inflation.2 However, this differential has been far from constant, and the series have diverged markedly at times. For instance, in the last quarter of 1981, CPI inflation ran about a full percentage point above PCEPI inflation, while during most of 2009 it ran an average of 0.35 ppts below PCEPI inflation (see figure 1). Why? In this Commentary, we discuss the differences in how the two measures are constructed,3 the reasons for their historical divergences, and the prospects for their relationship going forward. Based on a suite of forecasting models, we estimate that the differential going forward is likely to be close to its historical average, 0.30 ppts. This estimate might be useful, for example, in converting financial market expectations of CPI inflation into an implied projection for PCEPI inflation.
Differences in Construction
1. Scope. The CPI and PCEPI differ in coverage or scope; that is, the lists of items in the respective baskets differ. This difference in scope reflects different intended purposes, as there are conceptual differences between the two indexes. The CPI is designed to measure inflation in out-of-pocket spending by urban households, and thus its basket is representative of the goods and services that are purchased by urban consumers. In contrast, the PCEPI is designed to measure growth in the cost of the entirety of personal consumption expenditures in the national income and product accounts (NIPA). Thus, its scope is broader. In addition to the growth of prices related to out-of-pocket spending, the PCEPI must also account for growth in prices or costs of all goods and services purchased by entities such as governments, firms, or nonprofit institutions on behalf of the household sector. For instance, total spending on medical care includes both direct purchases of medical goods and services by consumers, as well as the spending on medical goods and services on behalf of households by Medicare or employer-provided health insurance companies. As another example, public schools provide education that is not paid for out-of-pocket. And the PCEPI also includes such things as the imputed costs of financial services that do not involve out-of-pocket spending. All told, about 25 percent of PCE spending is not captured by the CPI. It is worth noting that this broader scope comes with some disadvantages from a measurement perspective. The estimation of unobserved prices or costs can be quite challenging. And as Clark (2001) notes, because spending data for many items are available only on an annual basis, the BEA uses judgmental trends to estimate roughly20 percent of the PCEPI at a monthly frequency.
2. Expenditure weights. Partly because of this difference in scope, the CPI and PCEPI differ in expenditure weights (or the relative importance of various items in the indexes). In constructing an index number, which is effectively a weighted average, some price components get a heavier weight than others; but these weights differ across the two indexes. The broader scope of the PCEPI tends to lead to smaller weights on all of the items the two indexes have in common. Housing is a leading example. Both indexes use an owners’ equivalent rent approach to track housing cost inflation for homeowners,4 meaning that housing cost movements are driven by growth in market rents. Measured or estimated expenditures on housing comprise roughly 33 percent of out-of-pocket spending—thus housing has roughly a weight of 33 percent in the CPI; but housing is roughly 16 percent of total consumption—thus housing has roughly a weight of 16 percent in the PCEPI. Historically, rent inflation has tended to be at, or above, average inflation; thus rising rents have lifted CPI inflation above PCEPI inflation. Gasoline is another example of an item with heavier weight in the CPI than in the PCEPI. However, weights differ for other reasons, too. While expenditure weights in the CPI mainly derive from a survey of consumers, expenditure weights in the PCEPI typically derive from business surveys (such as the Census Bureau’s monthly retail surveys and its Quarterly Services Survey), and in some cases (such as alcohol) there is a notable discrepancy in weights, even after taking into account the differences in scope. One advantage of the PCEPI surveys is that they are far more timely, allowing more frequent updating of weights and thus, presumably, more accurately account for consumer substitution.
3. Formula. The formula difference is more technical. The CPI index is an average that is based, at the upper level of aggregation, on a Laspeyres formula.5 This means that the CPI implicitly assumes that the average household does not reduce its expenditure on housing in San Francisco so that it can purchase cheap bananas in Buffalo. The PCEPI is based on a Fisher-Ideal formula, which implicitly allows for the possibility of much more substitution. A Fisher formula generally results in a lower inflation estimate than a Laspeyres formula, even if the price changes used are identical. The details are fairly complicated and go beyond the scope of this paper. The bottom line is that, while both indexes account for substitution between goods (or services) when the relative prices of those items change, the PCEPI does this in a more timely and more thorough manner, allowing for substitution shifts across categories of goods.6 As Clark (2001) notes, estimates of this particular bias have been fairly stable at about +0.2 ppts (CPI inflation above PCEPI inflation) for decades. We believe that this is the most persistent source of the differential, although it may not be the dominant source in any given time period. If over the medium term, all price changes in the economy were approximately drawn independently from the same distribution, the differential between the two indexes would be driven by this formula difference. But they are not drawn from the same distribution. Many goods and services have persistent trends. For instance, many goods, such as home appliances and new vehicles, have actually fallen in price (once quality changes are taken into account) since 1980; many services, such as higher education, rent, and healthcare, have experienced large price gains since 1980.
4. Other. Finally, there are a number of less important differences between the indexes, such as the treatment of seasonal adjustment or the source of the price information. Usually the PCEPI just uses component indexes in the CPI, so the price data are identical. But for some goods and services, different source data are used. For instance, the CPI index for airline fares is based upon prices charged for air travel in a sample of routes; the PCEPI is instead based on passenger revenues and the total number of miles traveled by passengers. Also, as noted above, the BEA must estimate or impute prices for nonmarket goods. Historically, its imputation for financial services provided without charge resulted in a highly volatile price estimate (see Clark, 1999). Also, the BEA must estimate spending on households by nonprofit institutions such as religious and welfare organizations; it does not attempt to estimate the “total” value of those services, but rather uses their operating expenses as its measure of the value provided to people. For a cogent summary with some detailed examples, see BLS (2011).
5. Revisions. The nonseasonally adjusted CPI is almost never revised, so the seasonally adjusted CPI is essentially revised only to update seasonal adjustment factors; methodological improvements affect current and future values of the index, but are not applied to historical data.7 This feature of CPI data facilitates their use for indexing wages and government benefits, for indexing income tax brackets, and so on. In contrast, the BEA does not even produce a nonseasonally adjusted PCEPI and revises the PCEPI frequently and routinely. The initial PCEPI estimate is updated twice to reflect more complete data, and each year the PCEPI data for the previous three years are subject to revisions. Finally, with benchmark revisions of the NIPA occurring every five years, the PCEPI is also updated. To the fullest extent possible, methodological updates are applied to the entire history of the price index. These revisions can be quite notable (see, e.g., Clark, 1999 or Armen and Koenig, 2017). The analysis in this paper uses current (September 2019) vintage PCEPI data.
Historical Divergences
As noted above, the BLS does not retrospectively adjust the CPI’s history when methodological improvements are undertaken. However, the BLS provides a “research” series, the CPI-U-RS, which does retrospectively adjust CPI history in a methodologically consistent manner; in other words, this series approximates what the CPI would have been historically, had all current improvements been implemented starting in December 1978. While recent values of inflation as measured by the CPI-U-RS and the CPI-U are essentially the same, as one goes farther back in time it is better to compare inflation in the PCEPI to inflation in the CPI-U-RS, because the entire history of PCEPI inflation reflects current methodological improvements as well. Thus using the CPI-U-RS will be more helpful if one is interested in understanding how the CPI and PCEPI are likely to relate to one another going forward.8
Figure 1 depicts 12-month CPI-U-RS inflation, 12-month PCEPI inflation, and the inflation differential between the two, from December 1978 through September 2019. CPI inflation tends to exceed PCEPI inflation. Over this period, the average CPI–PCEPI inflation differential was +0.30 ppts.9 However, a differential of +1.0 ppts or more occurs on average once every 20 months. But CPI inflation does not always run above PCEPI inflation. For instance, during most of 1986 and into 1987, CPI inflation fell below PCEPI inflation, averaging a differential of about −0.4 ppts (CPI inflation below PCEPI inflation). More recently, during most of 2009, a similar shortfall occurred. In fact, over the entire period depicted in the figure, CPI inflation has fallen short of PCEPI inflation 20 percent of the time. A histogram of the monthly differential is provided in figure 2, which also denotes the median differential in orange.
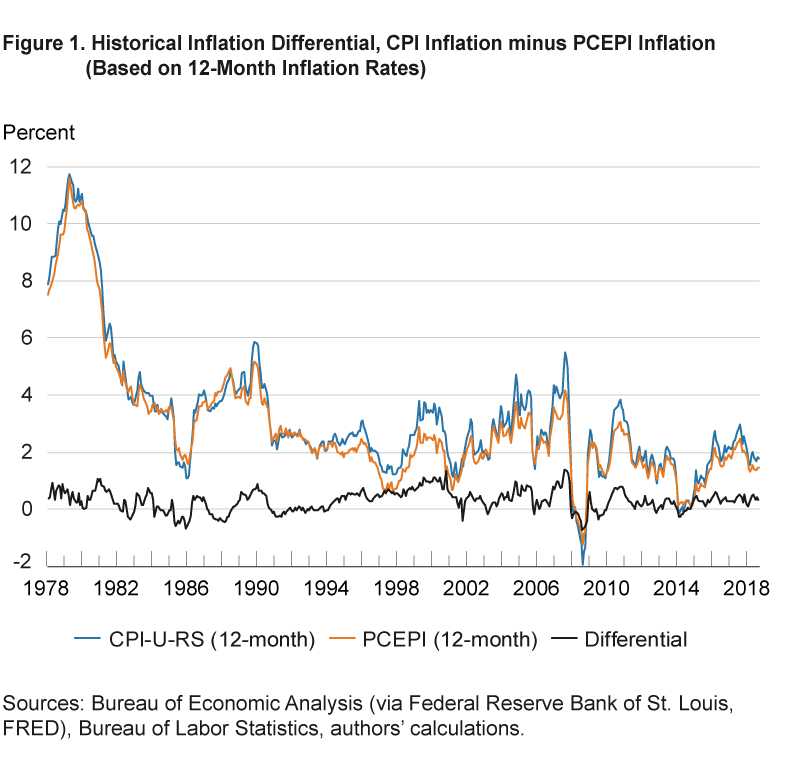
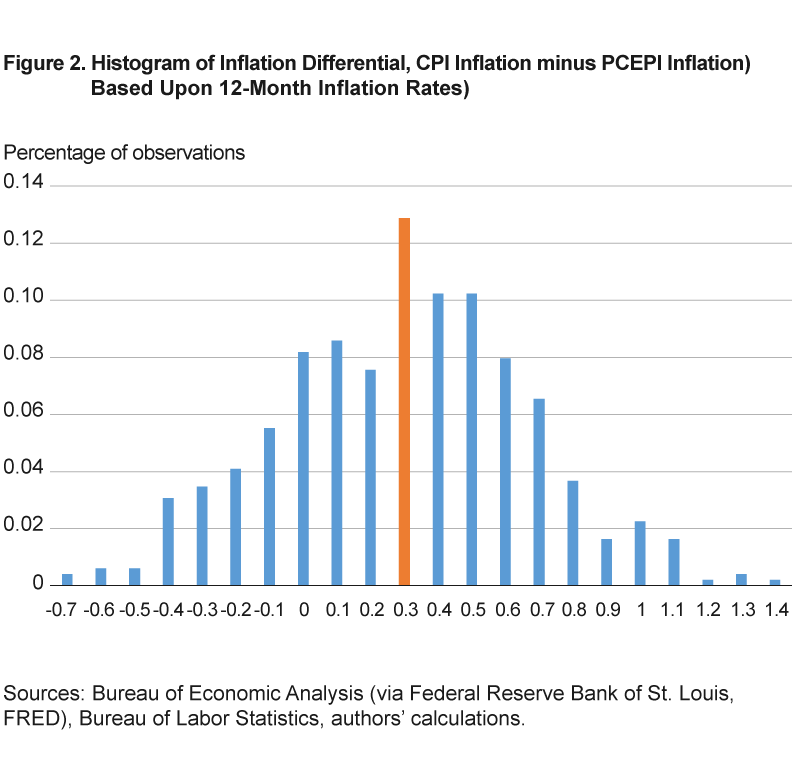
On a monthly basis, the BEA produces a document10 that decomposes the quarterly percentage differences between CPI inflation and PCEPI inflation into four components: scope, weight, formula, and “other.” (As reported by the BEA, a negative number means that PCEPI inflation is running below CPI inflation; in the figure, we switch the sign of the BEA numbers so as to be consistent with the rest of our article.) The sources of the differences vary significantly from quarter to quarter. To smooth the data, figure 3 below plots four-quarter moving averages of these components from 2003 onward. Typically, the weight effect and the scope effect are the dominant drivers of the differential (these enter with opposite signs, and their correlation is −0.54); formula (which is highly positively correlated with weight) is a distant third, and “other” is only occasionally important. On average, over the time period 2003 to 2019 in the figure, the differential was 0.27.
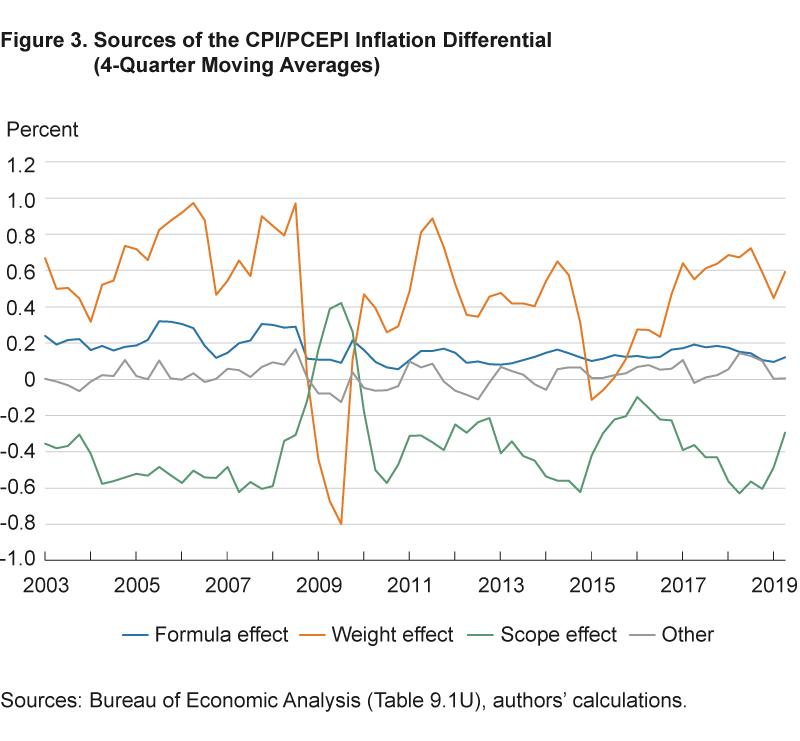
Around early 2009, we can see the source of the anomalous behavior of the CPI–PCEPI inflation differential mentioned above. Both the scope effect and the weight effect experienced unusual moves. The scope effect—which since 2003 has tended to push PCEPI inflation above CPI inflation—reversed sign and exerted a downward force on PCEPI inflation relative to CPI inflation. However, at the same time, the weight effect—which since 2003 has tended to cause PCEPI inflation to run below CPI inflation—also reversed sign, ultimately causing PCEPI inflation to run above CPI inflation.
What happened during this year to cause unusual moves in the weight effect and the scope effect? In 2008:Q4 and 2009:Q1, energy prices (and, in particular, gasoline) experienced a very large drop. This pushed both CPI inflation and PCEPI inflation lower, but since the CPI places a larger weight on energy than does the PCEPI, it pushed down CPI inflation more; thus the weight effect, which usually causes CPI inflation to run higher than PCEPI inflation, moved negative. (Gasoline price movements caused something similar to happen in 2014:Q4–2015:Q1.) Counterbalancing this to a modest extent, over 2008:Q4–2009:Q1, three items that are included in the PCEPI but excluded from the CPI—namely, financial services provided without payment, foreign travel by US residents, and nonprofit expenditures on behalf of households—fell notably. While these items usually exert an upward force on PCEPI inflation relative to CPI inflation, over this period they exerted a downward force.
It is not at all unusual for two of these components—energy, and financial services and insurance—to exert a strong influence on the differential. Indeed, if one uses a linear regression to explore the historical drivers of the differential, then one can account for 73 percent of the post-2000 variability in the differential using just these two components.11
Prospects Going Forward
Our previous discussion indicates that we can expect CPI inflation to generally exceed PCEPI inflation, but that short-lived reversals are also likely. In this section, we consider whether there are any longer-term trends that would suggest that the average differential will be different from the historical differential of 0.3 ppts. The answer to this question is of interest to analysts, for example, who wish to convert the implied CPI inflation forecasts from financial markets into their PCEPI inflation equivalents. In particular, should one simply remove 0.3 ppts from CPI inflation forecasts to convert them to their PCEPI inflation equivalents, or is there reason to believe that an alternative conversion factor, based upon forecasting models, is better?
Building upon the work of Hakkio (2008), we use a variety of reliable forecasting models, including survey-based forecasts, to address this question. Since an average of forecasts is often superior to even the forecast from the model with the best historical performance, we then compute a simple average of the forecasts from a handful of top-performing models. In the online appendix, we provide details about the specification of lesser-known models and a forecasting diagnostic, the root-mean-squared error (RMSE) of the forecast. Here, for brevity, we simply list the models we use, provide a forecast from each model, and then compute the average.
In general, we are more interested in longer-run trends of the CPI/PCEPI inflation differential than in short-term fluctuations. For our forecasting exercise, we calculate the longer-run trend using a five-year moving average of the differential. To get a sense of the variation in the longer-run trend, the centered moving average is plotted in black in figure 4 along with the quarterly differential, which is calculated as the quarterly annualized CPI inflation rate minus the quarterly annualized PCEPI inflation rate.12 At each point in time, we generate a forecast of the differential going forward and then compare it with the “true” future differential, which we take to be the five-year-ahead moving average. We evaluate forecasting accuracy over two sample periods, one from 2007:Q1 through 2019:Q3, and a longer sample from 1985:Q1 through 2019:Q3.13 We focus on quarterly rather than monthly data, since this allows us to include the estimates based upon the data underlying figure 3 and the Survey of Professional Forecasters.
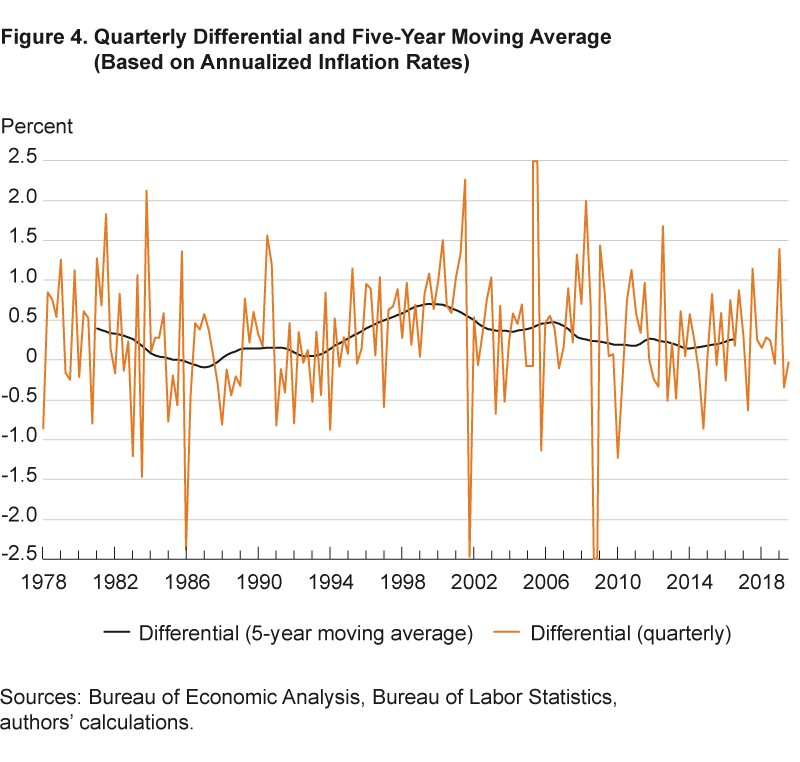
We consider the following eight approaches to forecast the differential.
1. We assume the forecast of the CPI inflation–PCEPI inflation differential is fixed at +0.2 ppt, corresponding to the Clark (2001) estimate of the formula bias.
2. We calculate the five-year/five-year-forward inflation differential that is implied from the median CPI inflation forecasts and the median PCEPI inflation forecasts over the next 5 years and the next 10 years in the quarterly Survey of Professional Forecasters. As of 2019:Q3 this differential was +0.21 ppts.
3. The third model uses a regression to estimate CPI inflation based on a constant and PCEPI inflation. We use only the last 20 years of data at any given time period, which is a way to allow coefficient estimates to change over time. We then convert these estimates into a forecast of the CPI–PCEPI inflation differential by using the current coefficient estimates, but inserting the “typical” value of 2 percent for PCEPI inflation; thus if β1 is the estimated constant and β2 is the estimated coefficient on PCEPI inflation, then the implied differential is given by(β1 + β2(2))−2. As of 2019:Q3, this forecast was +0.33 ppts.
4. We construct and forecast a price relative ratio; we then convert this forecast into an inflation differential forecast. A price relative is related to an inflation rate, but rather than being stated in terms of the percentage change of the price index, it is instead stated in terms of the ratio of the price index between one month and the next, namely P(t)/P(t−1). We form the ratio by placing the CPI price relative in the numerator, and the PCEPI price relative in the denominator. We take a five-year moving average of this ratio as the forecast, then convert that price ratio forecast into an inflation differential forecast. Its forecast in 2019:Q3 was +0.32.
5.,6. Our fifth and sixth models are “time-varying-parameter” models. These use PCEPI inflation to forecast CPI inflation, using a regression model whose coefficients smoothly change over time. These methods can be sensitive to outliers. Thus, owing to the undue influence of a handful of outliers for these specifications and our time period, each of these models is estimated on a data sample from which we remove the outliers from 2000:Q1 onward.14 The main difference between the two models is that model 5 only uses PCEPI inflation to forecast CPI inflation, while model 6 also includes a lag of CPI inflation in the model. The estimated coefficients are then used to derive an implied forecast of the inflation differential. In 2019:Q3, the two forecasts, respectively, were +0.29 and +0.46.
7. Our seventh model is a Bayesian vector autoregressive (VAR) model, which includes two lags of a four-quarter moving average of the CPI inflation–PCEPI inflation differential, and two lags of energy inflation. We use only the last 20 years of data at any given time period, which is a way to allow coefficient estimates to change over time. As of 2019:Q3, this model’s forecast was +0.23.
8. Our final forecasting model builds upon the four data series in figure 3. Because these data start in 2003, this model cannot be used in the forecast comparison. Despite the fact that we thus cannot assess its historical validity, we believe our “bottom-up” forecast based upon forecasts of the different components of the differential is still likely to be a fruitful addition to our set of forecasts. We estimate a separate regression model for each source, choosing the best univariate autoregressive-moving average (ARMA) model in each case. The current longer-run projections from each model are essentially equal to their historical averages. Summing these together, we obtain a predicted differential of +0.27 as of 2019:Q3.
Our forecast comparison results are reported in table 1. The most accurate forecasts over either the full sample or the post-2007 sample have been from the simplest model that says that the differential will be +0.2 ppts. In the post-2007 period, the SPF-based differentials have been fairly comparable in terms of forecast accuracy. In general, though, the forecasting performances across all of the models over the full sample have been fairly similar, as the RMSEs are quite comparable.
|
RMSE | Forecast as of 2019:Q3 | |
---|---|---|---|
|
post-2007 | post-1985 | |
Fixed at +0.2 ppts | 0.050 | 0.224 | 0.20 |
SPF implied differential | 0.054 | — | 0.21 |
Direct forecast | 0.168 | 0.313 | 0.33 |
Price relative-based | 0.131 | 0.243 | 0.32 |
Time-varying model 1 | 0.085 | 0.284 | 0.29 |
Time-varying model 2 | 0.126 | 0.250 | 0.46 |
Bayesian VAR | 0.211 | 0.323 | 0.23 |
Figure 3 ARMA | 0.27 | ||
Average |
|
|
0.29 |
What would these models predict for the differential going forward? Taking the average over all of these eight models results in a forecast of the CPI inflation–PCEPI inflation differential of +0.29 ppts as of 2019:Q3.15 The historical differential, +0.30, only very slightly overestimates this, and thus appears to be a solid conversion factor after all!
Conclusion
The CPI and PCEPI are two alternative measures designed to track inflation rates for households. They differ in construction, and because of this, they often give similar but slightly different signals about inflation. Usually CPI inflation runs above PCEPI inflation, but not always.
In this Commentary, we explain key differences between the two indexes, explore a historical episode of unusual divergence, and provide updated estimates of the expected differential going forward. Based on this analysis, we project that the differential over the next few years is likely to be close to its historical average, +0.29 ppts (CPI inflation above PCEPI inflation). This provides a useful rule of thumb for readers interested in converting one of these inflation rates to its alternative, roughly equivalent measure.
Footnotes
- In January 2012, the FOMC announced that “The Committee judges that inflation at the rate of 2 percent, as measured by the annual change in the price index for personal consumption expenditures, is most consistent over the longer run with the Federal Reserve’s statutory mandate.” Source: www.federalreserve.gov/newsevents/pressreleases/monetary20120125c.htm. Return
- Inflation is measured as percentage growth in the index. Return
- We can only provide a summary. For more details and discussion, see, e.g., Clark (2001) or McCully, Moyer, and Stewart (2007). Return
- The owners’ equivalent rent approach estimates the price change (or change in value) of the services yielded by a dwelling between one month and the next using the change in the observed market rents for similar types of dwellings. At present, “similar” is taken to mean “in the same neighborhood”; thus, if the market rent in a given neighborhood rises on average by 0.2 percent, the owners’ equivalent rent for owned homes in the neighborhood might be assumed to rise by 0.2 percent as well. For more details, see Diewert (2009). For a recent criticism of this “only the same neighborhood matters” approach, see Adams and Verbrugge (2020). Return
- Price indexes are generally constructed in two stages, first by computing average price movements for particular categories of goods and services (such as “rent in Cleveland”) and then by averaging over the price movements of those categories to form a national index. There are a number of different formulas that can be used to construct such averages. Return
- Since 2002, the BLS has produced a chained consumer price index (the C-CPI-U) that allows for such substitution, but the required expenditure data are available only with a time lag, and thus final estimates are released with a lag of about a year. Given its data sources, the BEA receives expenditure estimates much more often than does the BLS, so BEA expenditure weights can be updated more frequently. Return
- The BLS will occasionally revise the CPI to correct for data collection or processing errors. However, seasonal revisions can be notable; see Knotek and Zaman (2017). Return
- Despite continual research and improvements in each index, both are believed to be upward-biased estimates of the true rate of inflation facing consumers. Most recently, Moulton (2018) estimates that the CPI is upward-biased by 0.85 ppts, and that the PCEPI is upward-biased by 0.47 ppts. Return
- The distribution of divergences is nearly symmetric, so the median differential is nearly the same, +0.31 ppts. Return
- Table 9.1U. Reconciliation of Percent Change in the CPI with Percent Change in the PCE Price Index. This analysis recomputes the PCEPI by changing its method of computation, weights, and scope in a series of steps so that, in the end, it recomputes the CPI. This allows one to estimate the importance that each particular difference is responsible for. Return
- Our linear regression includes two lags of the differential, the contemporaneous effect of both energy inflation and financial services and insurance inflation, and one lag of each of these variables as well, to allow for a lagged influence. Energy inflation is the more important influence; on its own, it can account for 54 percent of the post-2000 variability in the differential. Return
- To eliminate noise, we compute our five-year moving average in a particular way; see the online appendix for details. We also restrict the scale of the figure to [−2.5, 2.5], which results in the truncation of three quarterly observations. Return
- This horizon does not necessarily correspond to any particular CPI forecast derived from other sources. We make this somewhat arbitrary choice because we believe that a 2.5-year horizon provides a more reliable read of the current longer-run trend than would a longer-horizon forecast, since—as professional forecasters know—longer-horizon forecasts often either simply revert to a historical mean (thus ignoring the recent data), or go off in a puzzling direction (owing to estimation errors). Return
- In particular, we use the RMVN method of Zhang, Olive, and Ye (2012) to remove bivariate outliers. Return
- We find it reassuring that the median forecast is nearly the same, +0.28 ppts. Return
References
- Adams, Brian, and Randal Verbrugge. 2020. “Location, Location, Structure-Type? Rent Inflation Differentials within Neighborhoods.” Unpublished manuscript, Bureau of Labor Statistics.
- Armen, Alan, and Evan F. Koenig. 2017. “Getting a Jump on Inflation.” Federal Reserve Bank of Dallas, Economic Letter, 12(9).
- Bureau of Labor Statistics. 2011. “Differences between the Consumer Price Index and the Personal Consumption Expenditures Price Index.” Bureau of Labor Statistics, Focus on Prices and Spending, Consumer Price Index: First Quarter 2011, 2(3).
- Clark, Todd E. 2001. “Comparing Measures of Core Inflation.” Federal Reserve Bank of Kansas City, Economic Review, Q2: 5–31.
- Clark, Todd E. 1999. “A Comparison of the CPI and the PCE Price Index.” Federal Reserve Bank of Kansas City, Economic Review, Q3: 15–29.
- Diewert, W. Erwin. 2009. “Durables and Owner-Occupied Housing in a Consumer Price Index.” In Price Index Concepts and Measurement, NBER, Studies in Income and Wealth, 70: 445–506. W.E. Diewert, J. Greenlees and C.R. Hulten (eds.), Chicago: University of Chicago Press.
- Hakkio, Craig S. 2008. “PCE and CPI Inflation Differentials: Converting Inflation Forecasts.” Federal Reserve Bank of Kansas City, Economic Review, 93(1): 51–68.
- Knotek, Edward S., and Saeed Zaman. 2017. “Nowcasting US Headline and Core Inflation.” Journal of Money, Credit, and Banking, 49(5): 931–968. https://doi.org/10.1111/jmcb.12401.
- McCully, Clinton P., Brian C. Moyer, and Kenneth J. Stewart. 2007. “A Reconciliation between the Consumer Price Index and the Personal Consumption Expenditures Price Index.” Survey of Current Business, November, 26–33.
- Moulton, Brent R. 2018. “The Measurement of Output, Prices, and Productivity: What’s Changed Since the Boskin Commission?” A Report of the Hutchins Center on Fiscal and Monetary Policy at Brookings. The Brookings Institution.
- Zhang, Jianfeng, David J. Olive, and Ping Ye. 2012. “Robust Covariance Matrix Estimation with Canonical Correlation Analysis.” International Journal of Statistics and Probability, 1(2): 119–136.
Suggested Citation
Janson, Wesley, Randal J. Verbrugge, and Carola Conces Binder. 2020. “The CPI–PCEPI Inflation Differential: Causes and Prospects .” Federal Reserve Bank of Cleveland, Economic Commentary 2020-06. https://doi.org/10.26509/frbc-ec-202006
This work by Federal Reserve Bank of Cleveland is licensed under Creative Commons Attribution-NonCommercial 4.0 International
- Share