Introduction to the Cleveland Fed Survey of Regional Conditions and Expectations (SORCE) Indexes
This District Data Brief introduces the Cleveland Fed Survey of Regional Conditions and Expectations (SORCE) indexes, which provide a timely summary of economic conditions in the Fourth Federal Reserve District. The indexes are based on responses to the Cleveland Fed SORCE, a survey of business and community leaders about regional economic conditions.
The views authors express in District Data Briefs are theirs and not necessarily those of the Federal Reserve Bank of Cleveland or the Board of Governors of the Federal Reserve System. The series editor is Harrison Markel.
The regional analysis group1 at the Federal Reserve Bank of Cleveland monitors the economy in the Fourth Federal Reserve District2 by tracking a variety of data and gathering information from people in the community. One of the ways we gather this information is the Cleveland Fed Survey of Regional Conditions and Expectations (SORCE), a survey of business and community leaders.** This survey generates valuable information on economic conditions that is more timely than most economic statistics, such as employment and output estimates. It also provides an opportunity for survey respondents to share their concerns and insights about how monetary policy is affecting “Main Street.” The information from this survey is one input that informs the Cleveland Fed’s contributions to the Beige Book and preparation for Federal Open Market Committee (FOMC) meetings.
This District Data Brief introduces the data we produce from SORCE. Gathering information from the community has been central to the Cleveland Fed since its founding, and in June 2014, we began systematically quantifying this information to produce the SORCE indexes. As shown below, the SORCE indexes have tracked more traditional sources of economic data effectively over the past 10 years. We are scheduled to start regularly releasing the SORCE indexes in January 2025. This will provide the public with a new set of timely measures of economic activity in the Fourth District.
The Survey
We survey business and community leaders eight times per year, timed to precede the FOMC meetings by roughly five weeks. Our survey covers the following topics: demand for goods and services, prices, employment, wages, planned capital expenditures, and near-term expectations. There are additional industry-specific questions that we ask regularly and special questions that cover timely topics. For example, in 2020, we surveyed firms about supply chain disruptions, as detailed in Dunn (2021).
We recruit survey respondents on an ongoing basis. Our respondents represent a wide variety of businesses and nonprofit organizations. The businesses range from small, single-location firms to Fortune 500 companies with a global footprint. Our survey sample has grown over time, with an average of more than 100 responses per survey round annually since 2019.
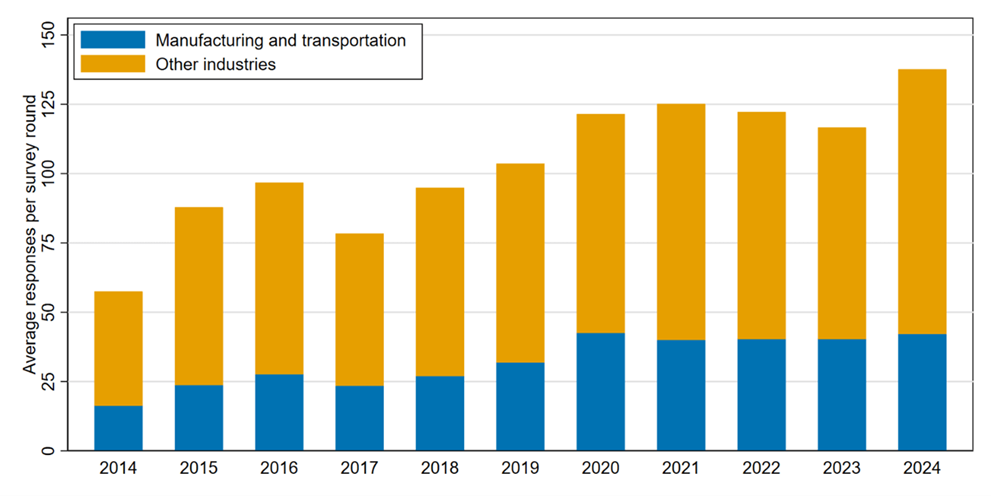
Source: Cleveland Fed.
We strive to have a sample that covers a broad range of industries (Table 1). However, our sample is intentionally designed to include a disproportionate number of firms in industries that tend to be leading indicators of economic turning points, such as construction and manufacturing. The three industries that accounted for the largest share of our sample in 2023 were manufacturing (27.4 percent), retail (19.6 percent), and construction and real estate (15.1 percent). We combine industries into two large sectors, “manufacturing and transportation” and “other industries” (defined in Table 1).
Table 1. Industry Composition of Survey Sample, 2023
Industries | Average responses per survey round | Percentage of responses |
Manufacturing and transportation | 40 | 34.5 |
Manufacturing | 32 | 27.4 |
Transportation | 8 | 7.1 |
Other industries | 77 | 65.5 |
Retail | 23 | 19.6 |
Construction and real estate | 18 | 15.1 |
Financial services | 13 | 11.3 |
Professional and business services | 10 | 8.7 |
Not elsewhere classified | 13 | 10.8 |
Total | 117 | 100.0 |
Source: Cleveland Fed.
There have been several meaningful changes to our survey over time. In March 2016, we standardized the core questions (those which all respondents are asked) and added core questions about changes to wages and the firm’s expectations for demand, employment, and costs in the months ahead. At that time, we began using a web-based survey for all sectors, though we continue to interview a few people by phone. We began surveying members of our Business Advisory Councils once a quarter in March 2016 and expanded that to every survey round in March 2018.
The core questions in our survey ask about changes in the last two months. The response can be one of five levels or “Not applicable.” For example, the question about demand is
How has customer demand for your firm’s products or services changed in the last two months?- Greatly increased
- Increased
- No change
- Decreased
- Greatly decreased
- N/A
The questions about expected changes refer to “…the remainder of the current quarter and into the next quarter.” For example, the question about the expected change in staffing levels is
How do you expect your staffing levels to change over the remainder of the current quarter and into the next quarter?- Greatly increase
- Increase
- No change
- Decrease
- Greatly decrease
- N/A
In addition to multiple-choice questions, the survey has open-response questions for each topic that enable respondents to share details or their related thoughts. For the full text of our core survey questions, please see the online Appendix of this District Data Brief.
The Cleveland Fed Survey of Regional Conditions and Expectations
The SORCE indexes are derived from our core SORCE questions and summarize economic conditions as captured by this survey. These indexes are the difference between the percentage of the sample reporting increases and the percentage reporting decreases, which is often called a diffusion index. Our indexes range from −100 percentage points (everyone reports decreases) to 100 percentage points (everyone reports increases). An index of zero points indicates that there has been no change: the percentage reporting increases is offset by the percentage reporting decreases. We seasonally adjust our indexes and calculate their standard errors. For additional methodological details, please see the Appendix.
A number of other Federal Reserve Banks publish similar indexes from business surveys about economic conditions. Examples include the Chicago Fed’s Survey of Economic Conditions, the Dallas Fed’s Texas Business Outlook Surveys, the Kansas City Fed’s Manufacturing Survey, the New York Fed’s Empire State Manufacturing Survey, the Philadelphia Fed’s Business Outlook Surveys, and the Richmond Fed’s Fifth District Surveys. These examples are all monthly data, while the SORCE indexes have two data points per quarter.
Some SORCE indexes exclude some industries. For example, the financial services and staffing industries are excluded from the planned capital expenditures index because these industries were not surveyed about these expenditures prior to March 2016. Our prices and nonlabor costs index also excludes the financial services industry. The responses from the financial services industry to the relevant survey questions tend to be closely related to the federal funds rate, and we have found that excluding this industry helps our indexes track price and cost changes more effectively.
Like all survey estimates, our indexes have margins of error.3 For example, the 90 percent margin of error of the total business conditions index averaged +/−12 percentage points in 2023 (Table 2). Because the margins of error are large relative to the typical round-to-round change in the indexes, it is best to ignore small round-to-round changes and focus on large changes or trends over a few rounds. This is especially true for the sector-specific indexes, which have larger margins of error than the corresponding total indexes.
Table 2. Average Margin of Error of Cleveland Fed SORCE Indexes (Percentage Points), 2023
Index | Total | Manufacturing and transportation | Other industries |
Planned capital expenditures | 9.6 | 15.3 | 12.7 |
Business conditions | 12.0 | 21.6 | 14.7 |
Expected business conditions | 11.1 | 19.5 | 13.8 |
Employment | 9.7 | 18.3 | 11.5 |
Wages | 8.2 | 13.8 | 10.5 |
Nonlabor input costs | 10.7 | 19.2 | 12.9 |
Output prices | 11.3 | 20.3 | 13.6 |
Note: 90 percent margin of error based on standard error of the index and the t distribution. Source: Cleveland Fed.
Figure 2 shows the total and sector-level indexes for seven topics: planned capital expenditures, business conditions, expected business conditions, employment, wages, output price, and nonlabor input costs. These indexes respond as one would expect to changes in economic conditions. For example, the indexes reflected the dramatic economic shocks of the COVID-19 pandemic. Following the onset of the pandemic, all the indexes dropped sharply. Aside from the nonlabor input costs index, the total indexes reached their lowest points in series history during the first half of 2020.4 As the economy recovered from the pandemic from March 2021 through March 2022, all but one of the total indexes reached their peaks in series history. The exception, the planned capital expenditures index, was also high during this period: this index reached its second- and third-highest levels during the summer of 2021.
Within topics (for example, planned capital expenditures, business conditions, and employment), the “manufacturing and transportation” and “other industries” indexes tend to move in similar ways.5 However, there are periods in which the sector-level indexes diverge, such as when the oil price drop in early 2015 slowed oil and gas exploration. This slowdown affected manufacturing and transportation firms more dramatically than it affected firms in other industries. While the manufacturing and transportation sector’s business conditions index was below zero (indicating falling demand) in 11 of the 14 survey rounds from February 2015 through September 2016, the other industries’ business conditions index remained above 10 points (indicating rising demand) throughout this period.
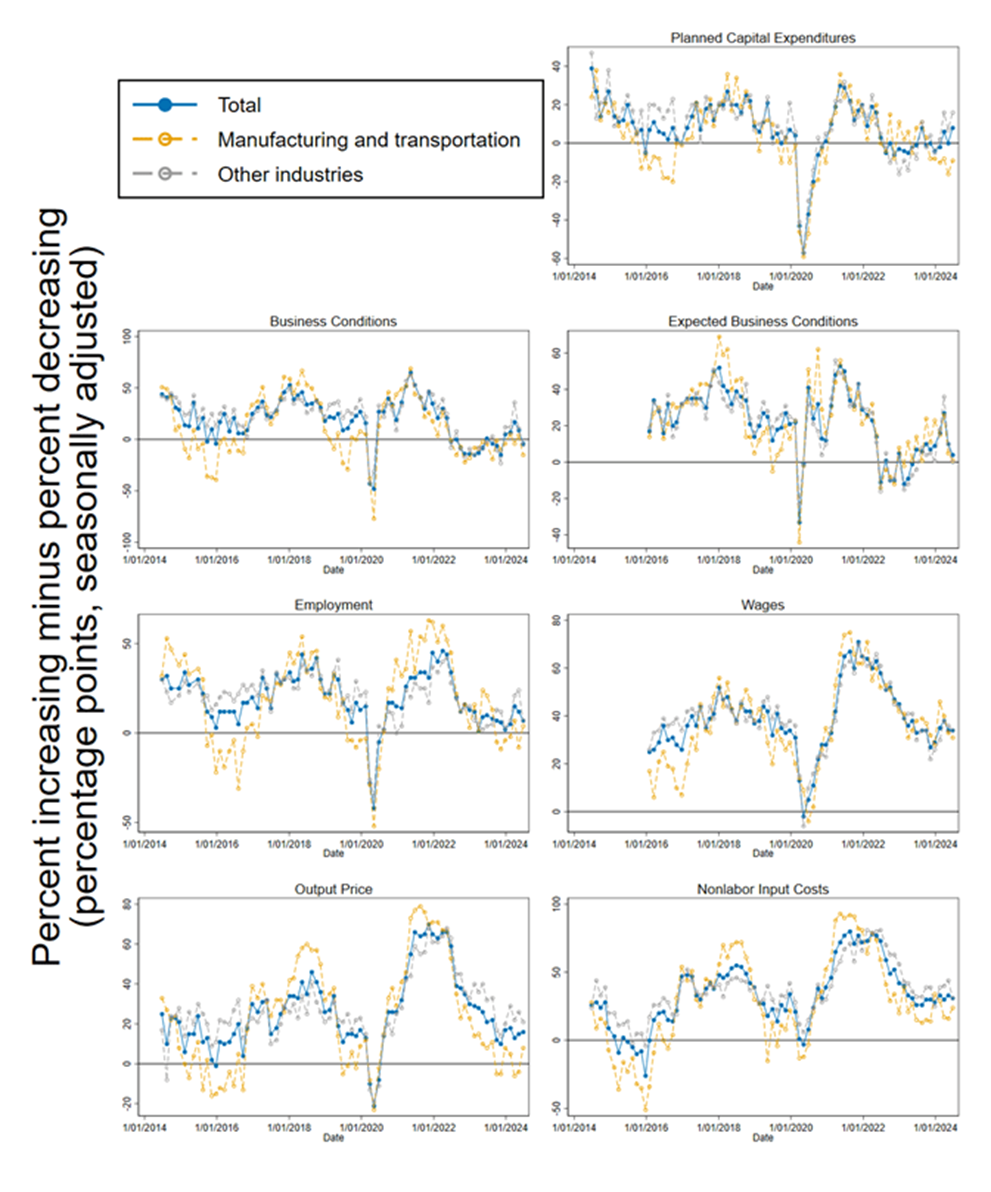
Source: Cleveland Fed.
Validating the Cleveland Fed SORCE Indexes
As shown in Figure 2 above and discussed in the prior section, the SORCE indexes responded as would be expected to the oil price shock of 2015 and to the COVID-19 recession and rapid recovery. This suggests that our indexes have value as timely indicators of economic conditions. In this section, we evaluate our indexes more rigorously by using regressions to test whether our indexes are significantly associated with changes in relevant economic data, including output growth, employment growth, and price inflation. These results confirm that our indexes have effectively tracked economic data over the past 10 years and provide some insights on how the indexes should be interpreted.
These regressions are akin to research that has shown that the information embodied in the Beige Book can be used to improve nowcasts of gross domestic product (GDP) and US employment (Armesto et al., 2009) and forecast recessions (Filippou et al., 2024). That research uses text analysis to quantify the information from the published text of the Beige Book. Here, we use our indexes to quantify the information from our survey.
In most of our regressions, the dependent variable is the annualized growth rate of a standard economic statistic. The exceptions are the regressions of the change in the unemployment rate on our employment index—here, the dependent variable is the one-month change in the unemployment rate. For outcomes with a quarterly frequency, we convert our indexes to quarterly data. For outcomes with a monthly frequency, we interpolate between months to estimate the level of the outcome at the time of our survey and one month ahead to get a monthly change at the time of our survey. We do this because we have found that our indexes were more related to changes in the past month than to changes in the past two months. This suggests that, while the survey asks people about changes over the past two months, their responses are more reflective of changes in the past month. The Appendix provides additional details on the regression methodology.
For each outcome and index pair, we estimate two regressions. The first is a simple bivariate regression of the outcome on the index and a constant. This tests whether the index is associated with the outcome. The second regression adds a one-period lag of the dependent variable for most outcomes, and it adds the level of the unemployment rate in the prior month for models of the change in the unemployment rate. This tests whether the relationship between our index and the outcome holds when accounting for autocorrelation in the outcome.
Table 3 summarizes the regression results (the full results are included in Table A1 in the Appendix). Our indexes are significantly correlated with the relevant economic outcomes, with one exception that I will discuss in the next paragraph. These results demonstrate that the SORCE indexes have successfully tracked economic conditions over the last 10 years. The only case in which adding the lagged outcome changes the association to insignificant is the association between the employment index and the District employment growth rate.6 The District’s month-to-month employment growth rate tends to be much more volatile than our employment index. While the employment index misses month-to-month volatility, it accurately reflects the trend in employment growth.
Table 3. Summary of Associations between the Cleveland Fed SORCE Indexes and Related Economic Data
Index and outcome | Is association statistically significant? | |
Index alone | With lagged outcome | |
a. Business conditions index | ||
Ohio real gross state product growth rate | No | No |
US real gross domestic product growth rate | Yes | Yes |
b. Employment index | ||
Change in District unemployment rate | Yes | Yes |
District employment growth rate | Yes | No |
c. Wages index | ||
US employment cost index growth rate | Yes | Yes |
Nominal US average hourly earnings growth rate | Yes | Yes |
d. Planned capital expenditures index | ||
US fixed investment growth rate | Yes | Yes |
e. Output price index | ||
US core producer price index inflation rate | Yes | Yes |
US core personal consumption expenditures inflation rate | Yes | Yes |
Note: See Table A1 in Appendix for the detailed regression results.
Source: Cleveland Fed.
The only index–outcome pair that is not significantly associated is the business conditions index and the quarterly growth rate of Ohio’s real gross state product (GSP). However, the business conditions index is significantly associated with the quarterly growth rate of real US GDP. One reason that our business conditions index may have a stronger association with that national growth rate than with the Ohio growth rate is that respondents are surveyed about firm-wide conditions, and many respondents are at firms with national or global footprints. Another possible explanation for the stronger association with the national growth rate is that Ohio’s GSP growth rate tends to be more volatile than the national GDP growth rate. Our business conditions index is also less volatile than Ohio’s GSP growth rate. This suggests that it is best to think of this index as a measure of growth trends, rather than a measure of contemporaneous growth rates.
Another insight about how the indexes should be interpreted comes from the pair of inflation regressions. Because the output price index is related to both producer price inflation and consumer price inflation, this index should be interpreted as a mixture of these two types of inflation. This reflects the fact that our sample includes both business-to-business and business-to-consumer firms.
Summary
The Cleveland Fed SORCE indexes comprise one input that informs the Cleveland Fed’s contribution to the Beige Book and preparation for FOMC meetings. The indexes provide information about a variety of economic conditions, including demand, labor markets, and inflation. Our indexes are significantly associated with changes in related economic data, a finding which confirms that these timely indexes provide valuable information. We look forward to releasing our indexes in the future so that the public has the opportunity to harness this information.
Footnotes
- Thanks to Jayme Gerring for assisting with this District Data Brief. Return to 1
- The Fourth District covers Ohio, western Pennsylvania, eastern Kentucky, and the northern panhandle of West Virginia. Return to 2
- Note that our survey sample is not a random sample. Return to 3
- The nonlabor input costs index reached its lowest point in December 2015 after falling oil prices triggered a slowdown in oil and gas extraction that led to reductions in steel prices. Return to 4
- The correlations between the two sectors’ indexes range from 0.54 (employment) to 0.79 (nonlabor input costs) across topics. Return to 5
- While the regression coefficient is statistically insignificant at the 5 percent level, it is significant at the 10 percent level. Return to 6
Appendix
Seasonal Adjustment
In August each year, we re-estimate seasonal factors and use the new factors to revise the most recent five years of data. We use seasonal-trend locally weighted scatterplot smoothing (commonly referred to as STL) to estimate additive seasonal factors. STL can produce poor estimates of seasonal factors at the beginning and end of the series. To avoid this, for the first 12 survey rounds (the surveys collected from June 2014 through November 2015), we set the seasonal adjustment equal to the seasonal factor from eight rounds (one year) later. Similarly, for the 12 most recent survey rounds, we set the seasonal adjustment equal to the seasonal factor from eight rounds (one year) earlier. We then assume that the seasonal factors for future survey rounds through the following July are equal to the seasonal factor from the same period in the most recent year.
We use these seasonal factors to adjust the percentages of responses that are “increasing” and “decreasing” to get per_up_sa’ and per_down_sa’, respectively. With additive seasonal factors, it is possible for these seasonally adjusted percentages to be below zero or above 100. After seasonal adjustment, we restrict each of these percentages to be in the range of 0 through 100 by setting values below zero equal to zero and values above 100 equal to 100. If per_up_sa’ + per_down_sa’ >100, we rescale these percentages by 100/(per_up_sa’ + per_down_sa’) and round them to integers. This gives the final seasonally adjusted percentages per_up_sa and per_down_sa. The seasonally adjusted index is ind_sa = per_up_sa − per_down_sa. The seasonally adjusted percentage of responses that are “no change” is per_same_sa = 100 − per_up_sa − per_down_sa.
This process produces a set of internally consistent, seasonally adjusted estimates of an index and the associated percentages of respondents reporting up, down, and no change.
Validation Regression Methodology
For the regressions with quarterly outcomes, we create quarterly indexes by taking weighted averages of the indexes in the survey rounds that overlap with the quarter, where the weight is determined by how many of the rounds’ days fall in the quarter.
Define the date as ; the last day of quarter q as ; and the average response date of survey s as . Then , with the weight for survey s in quarter q defined as
For the regressions where each period is a survey round, we interpolate between monthly outcome data to estimate the value on the average collection date of the survey round. Because monthly employment data are measured for the pay period that includes the twelfth of the month, we treat the published data (both employment and price index) as though it is for the twelfth of the month. The formula for the level of the variable is
where t indexes the month and is the outcome variable for the month that includes the average collection date of survey round s if that date falls before the twelfth of the month, or the month that follows the month of the average collection date for survey round s if that date falls on or after the twelfth of the month.
Our District payroll employment estimate includes employment estimates for several metro areas, and metro-level employment estimates have a history of large revisions (Elvery and Vecchio, 2014). Therefore, we use the District payroll employment data through September 2023, the latest month fully benchmarked by the Bureau of Labor Statistics (BLS). For all other outcomes, we use the latest data available as of June 5, 2024.
Each regression excludes a small number of outliers. Most of the outliers were in 2020 and 2021, when the COVID-19 pandemic led to rapid economic changes and both our indexes and the outcome data were atypical. The results of several of the models are sensitive to the inclusion of outliers, and the results excluding outliers better represent the typical relationship between our indexes and the outcome variables.
To reduce subjectivity, we define outliers using the stata command rreg, which estimates regressions that are robust to outliers. An observation is treated as an outlier if it has a Cook’s distance greater than 1 or if rreg sets the observation’s weight below 0.5, which indicates that the absolute value of the observation’s residual is far from the median absolute residual. This method identifies two to five outliers in the regressions with quarterly data and four to nine outliers in the regressions with survey-round data.
Validation Regression Results
Table A1 provides the results for the regressions summarized in Table 3 above. The dependent variable for the District unemployment rate regression is the one-month change in the District’s unemployment rate (in percentage points). All other dependent variables are annualized one-period growth rates (as percentages).
Table A1. Regressions of Changes in Economic Data on the Cleveland Fed SORCE Indexes
Dependent variable (periods) | Index alone | With lagged outcome |
a. Ohio real gross state product growth rate (quarters) | ||
Conditions index | 0.0266 | 0.0217 |
(0.0212) | (0.0217) | |
One-period lag of GSP growth rate | 0.1680 | |
(0.1669) | ||
Observations | 34 | 34 |
R-squared | 0.05 | 0.08 |
b. Real US gross domestic product growth rate (quarters) | ||
Business conditions index | 0.0393*** | 0.0330** |
(0.0137) | (0.0144) | |
One-period lag of GDP growth rate | 0.1890 | |
(0.1460) | ||
Observations | 35 | 35 |
R-squared | 0.20 | 0.24 |
c. District employment growth rate (survey rounds) | ||
Employment index | 0.0616*** | 0.0188* |
(0.0111) | (0.0108) | |
One-period lag of employment growth rate | 0.1266*** | |
(0.0192) | ||
Observations | 63 | 63 |
R-squared | 0.33 | 0.61 |
d. Change in District unemployment rate (survey rounds) | ||
Employment index | -0.0038*** | -0.0032*** |
(0.0011) | (0.0010) | |
One-period lag of unemployment rate | -0.0739*** | |
(0.0156) | ||
Observations | 68 | 68 |
R-squared | 0.16 | 0.37 |
e. US employment cost index (ECI) growth rate (quarters) | ||
Wages index | 0.0628*** | 0.0502*** |
(0.0113) | (0.0058) | |
One-period lag of ECI growth rate | 0.0614*** | |
(0.0065) | ||
Observations | 32 | 32 |
R-squared | 0.50 | 0.88 |
f. Nominal US average hourly earnings (AHE) growth rate (survey rounds) | ||
Wages index | 0.0502*** | 0.0618*** |
(0.0146) | (0.0164) | |
One-period lag of AHE growth rate | -0.1319 | |
(0.0896) | ||
Observations | 63 | 63 |
R-squared | 0.16 | 0.19 |
g. US fixed investment growth rate (quarters) | ||
Planned capital expenditures index | 0.2113*** | 0.1677*** |
(0.0488) | (0.0562) | |
One-period lag of fixed investment growth rate | 0.2173 | |
(0.1464) | ||
Observations | 37 | 37 |
R-squared | 0.35 | 0.39 |
h. US core producer price index (PPI) inflation rate (survey rounds) | ||
Prices index | 0.1067*** | 0.0813*** |
(0.0108) | (0.0182) | |
One-period lag of core PPI inflation rate | 0.1847* | |
(0.1075) | ||
Observations | 73 | 73 |
R-squared | 0.58 | 0.60 |
i. US core personal consumption expenditures (PCE) inflation rate (survey rounds) | ||
Prices index | 0.0621*** | 0.0238*** |
(0.0074) | (0.0085) | |
One-period lag of core PCE inflation rate | 0.5562*** | |
(0.0877) | ||
Observations | 73 | 73 |
R-squared | 0.50 | 0.68 |
Note: Standard errors in parentheses. Asterisks indicate level of statistical significance. *** = 1 percent, ** = 5 percent, and * = 10 percent.
Sources: Cleveland Fed, Bureau of Economic Analysis, BLS (via Haver Analytics).
**[03.21.2025. This article has been modified to correct the naming of the survey. An earlier draft of this District Data Brief referred to the survey as the “Summary of Regional Conditions and Expectations.” The survey is among the inputs that inform the Cleveland Fed's Beige Book contribution. This overrides the previous change in survey nomenclature added on 12.17.24.]
References
- Armesto, Michelle T., Rubén Hernández‐Murillo, Michael T. Owyang, and Jeremy Piger. 2009. “Measuring the Information Content of the Beige Book: A Mixed Data Sampling Approach.” Journal of Money, Credit and Banking 41 (1): 35–55. https://doi.org/10.1111/j.1538-4616.2008.00186.x.
- Dunn, Julianne. 2021. “Covid-19 and Supply Chains: A Year of Evolving Disruption.” Cleveland Fed District Data Brief. Federal Reserve Bank of Cleveland. https://doi.org/10.26509/frbc-ddb-20210226.
- Elvery, Joel A., and Christopher Vecchio. 2014. “Which Estimates of Metropolitan-Area Jobs Growth Should We Trust?” Economic Commentary, no. 2014-05 (April). https://doi.org/10.26509/frbc-ec-201405.
- Filippou, Ilias, Christian Garciga, James Mitchell, and My T. Nguyen. 2024. “Regional Economic Sentiment: Constructing Quantitative Estimates from the Beige Book and Testing Their Ability to Forecast Recessions.” Economic Commentary, no. 2024-08 (April). https://doi.org/10.26509/frbc-ec-202408.
Suggested Citation
Elvery, Joel A. 2024. “Introduction to the Cleveland Fed Survey of Regional Conditions and Expectations (SORCE) Indexes.” Federal Reserve Bank of Cleveland, Cleveland Fed District Data Brief. https://doi.org/10.26509/frbc-ddb-20241125
This work by Federal Reserve Bank of Cleveland is licensed under Creative Commons Attribution-NonCommercial 4.0 International
- Share
Survey of Regional Conditions and Expectations Indicator
The Cleveland Fed Survey of Regional Conditions and Expectations (SORCE) indexes provide a timely summary of economic activity in the Federal Reserve’s Fourth District. The indexes are based on responses to the Cleveland Fed SORCE, a survey of business and community leaders about regional economic conditions.