The Effect of Higher Financing Costs on Job Openings and Online Job Postings
The views authors express in Economic Commentary are theirs and not necessarily those of the Federal Reserve Bank of Cleveland or the Board of Governors of the Federal Reserve System. The series editor is Tasia Hane. This paper and its data are subject to revision; please visit clevelandfed.org for updates.
Introduction
From the outset of the COVID-19 pandemic until the spring of 2022, vacancies as measured by job openings and online job postings increased substantially. From a policy perspective, a change in the number of vacancies is important because it is one of the determinants of a common measure of labor market tightness: the ratio of vacancies to unemployed workers. Holding the number of unemployed workers constant, labor market tightness increases if the number of vacancies increases. In a tight labor market, workers can bargain for higher wages, putting upward pressure on wage growth (Domash and Summers, 2022; Brunow et al., 2022; and Jordà et al., 2022) and on inflation (Jordà et al., 2022).
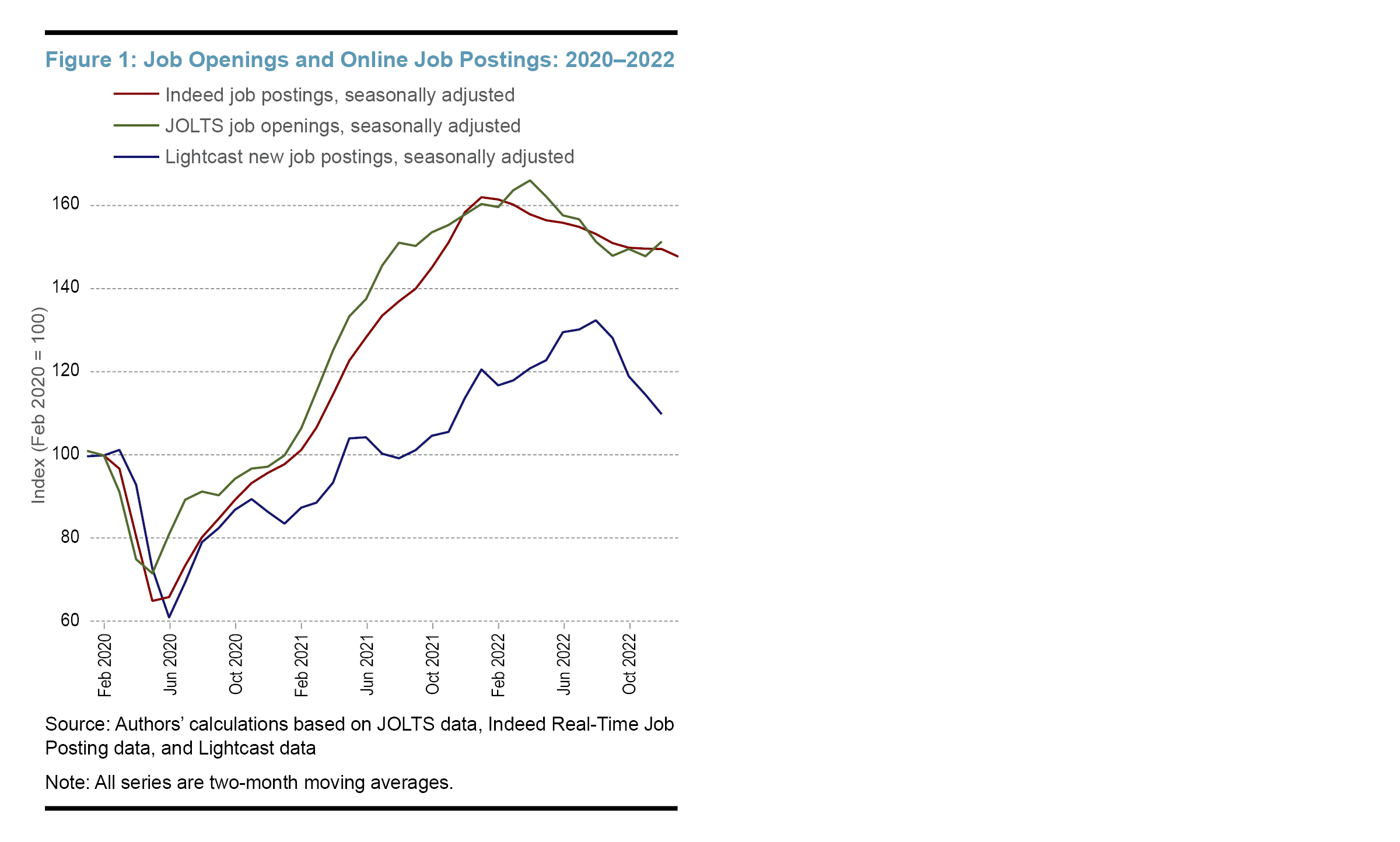
In response to elevated inflation, the Federal Open Market Committee (FOMC) started raising interest rates in March 2022, naming as one of its objectives the rebalancing of the labor market so that supply and demand of labor could better align, helping to bring inflation down (Powell, 2022). As interest rates began to increase, the upward trend in vacancies started to turn downward, and, as shown in Figure 1, over the last few months there has been a sustained decline in vacancies.1 For instance, from April 2022 to December 2022, the two-month moving average of the number of JOLTS job openings declined by 9 percent, (or, as shown in Figure 1, from 66 percent above its level in February 2020 to 51 percent above it ). Similarly, from its peak in the summer of 2022 to December 2022, the two-month moving average of online job postings from Lightcast declined by 17 percent (from 32 percent above its level in February 2020 to 10 percent above it). While previous work suggests that tighter monetary policy decreases labor demand (Garibaldi, 1997; Petrosky-Nadeau, 2014), the lags with which monetary policy affects real activity make it difficult to assess whether the recent declines in vacancies are a response to monetary policy tightening or simply arise from the natural evolution of the labor market. As such, the goal of this Economic Commentary is to answer whether the recent declines in vacancies are, at least in part, driven by monetary policy tightening. Answering this question is challenging since changes in monetary policy occur at the national level, thus limiting the sources of variation we can use to explain changes in labor demand. However, its answer is crucial to understanding whether the monetary policy tightening cycle that started in early 2022 is already influencing the labor market and, if so, to what degree.
To overcome this challenge, we propose two different approaches in this Economic Commentary. The first approach uses variation in industry exposure to monetary policy tightening to test whether industries more exposed to higher financing costs have seen larger declines in vacancies since March 2022.2 The second approach uses variation coming from the differential effects that monetary policy has on borrowing costs (as proxied by mortgage rates) across states to test whether states in which monetary policy tightening increases borrowing costs more than in other states have seen larger declines in vacancies since March 2022. We find that the declines in vacancies observed during the second half of 2022 are consistent with the effects we would expect from monetary policy tightening, suggesting that monetary policy is responsible in part for the cooling of the labor market in recent months when measured by vacancies.
Have Vacancies Declined Further in Industries That Were More Exposed to Higher Financing Costs?
Our first approach uses variation in how exposed different industries were to financial conditions and monetary policy tightening in early 2022 and correlates this level of exposure with the relevant industry’s change in vacancies since March 2022. We define the industry’s exposure to tighter financial conditions based on the industry’s assets and liabilities as of the first quarter of 2022. We use data from the US Census Bureau’s Quarterly Financial Report (QFR)3 at the industry level and present two different proxies of exposure.
For our first proxy, we focus on the ratio of the industry’s current liabilities (that is, the industry’s financial obligations due within a year) to current assets (that is, the industry’s assets that could be converted to cash in less than a year) as of 2022:Q1.4 The idea behind using this ratio as a proxy is that firms with higher ratios will be more likely to roll over their debt that is coming due because these firms have fewer available resources to use for debt repayment. Having to roll over their debt when monetary policy is tighter implies that the cost of servicing their debt will increase, reducing the resources available to initiate new projects or continue current ones and potentially reducing their labor demand. This channel is consistent with the findings in Cantor (1990) that show that a high-leverage firm’s employment and investment are more sensitive to the firm’s cash flows and that cash flow at a high-leverage firm is heavily affected by changes in the interest expenses the firm faces.
For our second proxy, we focus instead on the ratio of total liabilities relative to total assets during the first quarter of 2022, a measure of how much debt the industry has relative to what the industry is worth. Here, the rationale for the proxy is different than for the first. Firms that have higher debt levels might find more limitations in accessing credit when monetary policy tightens because their debt levels might limit the availability of credit (if monetary policy tightening makes firms more likely to face a hard borrowing limit) or its affordability (if monetary policy tightening makes the marginal cost of borrowing increase more for firms with more leverage). Both channels would be consistent with the findings of Hu (1999) and Ghosh and Ghosh (2006) that show that monetary contractions reduce the growth of investment more for highly leveraged firms.
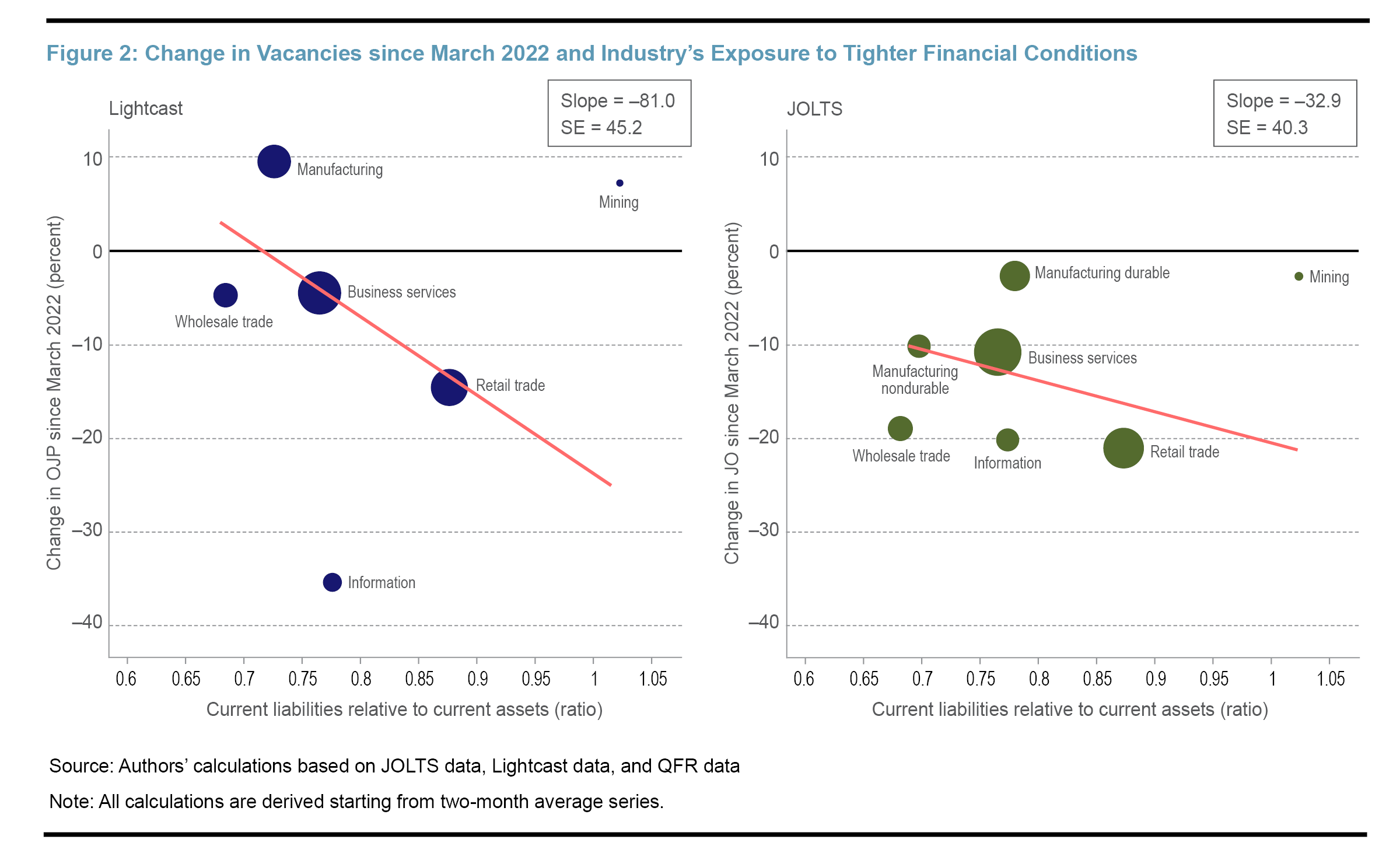
Figure 2 focuses on our first proxy, showing the relationship between the ratio of current liabilities to current assets and the change in job openings and online job postings from March to December 2022. Both panels of the graph depict the same idea but use different measures of vacancies. The x-axis shows the industry’s ratio of current liabilities to current assets. The y-axis shows the industry’s change in the seasonally adjusted level of new online job postings from Lightcast (left panel) and the seasonally adjusted level of job openings from JOLTS (right panel).
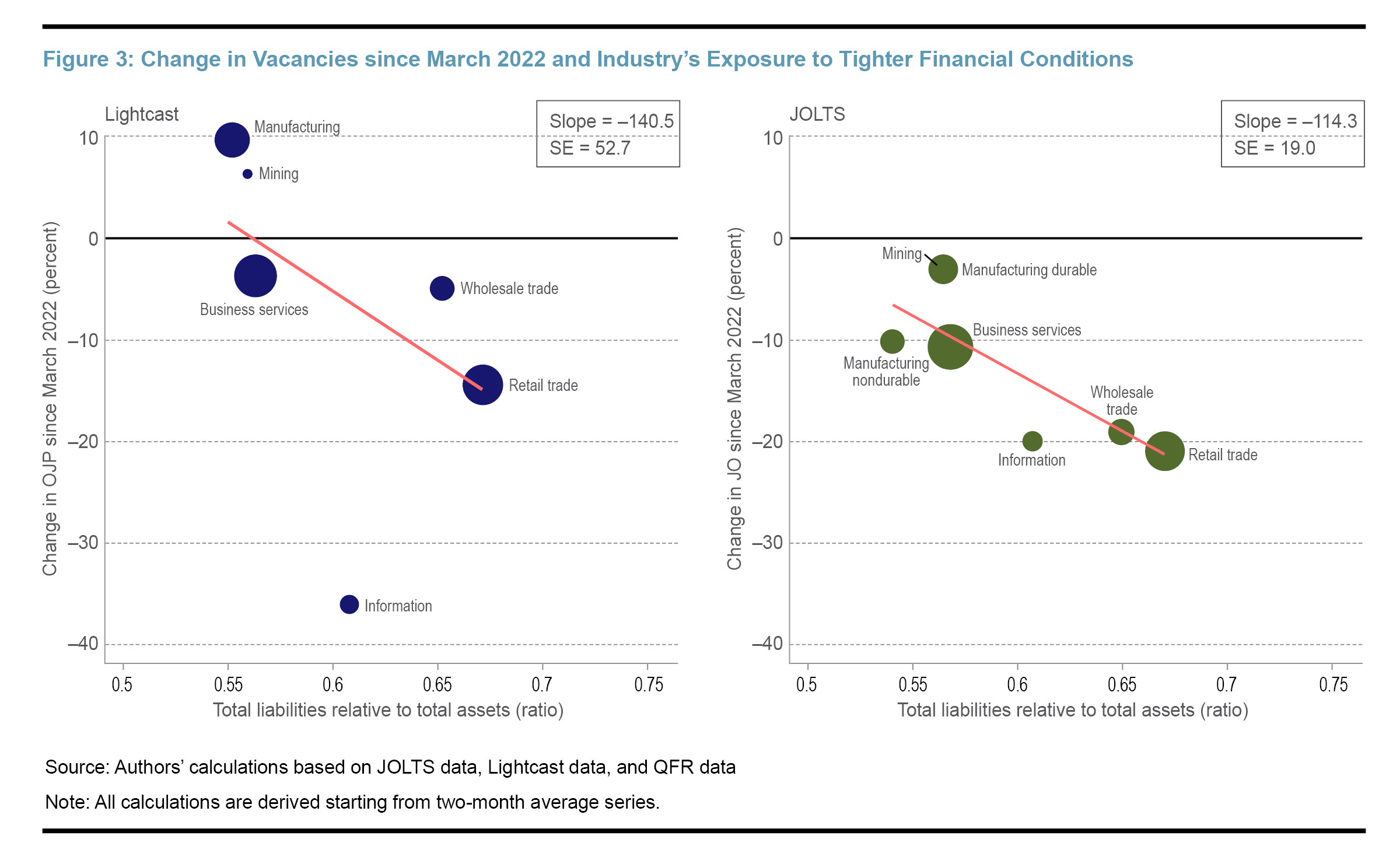
Figure 3 uses our second proxy. The x-axis here is the industry’s ratio of total liabilities to total assets, while the y-axis in each panel is identical to the ones shown in Figure 2.
The results suggest that industries more exposed to tighter financial conditions (that is, industries with a higher ratio of current/total liabilities to current/total assets in the first quarter of 2022) have seen larger declines in vacancies from March 2022 until December 2022.5 This is true both for online job postings (left) and job openings (right). This negative relationship is stronger when we use the ratio of total liabilities to total assets as a proxy for exposure to tighter financial conditions. Therefore, from our first exercise, we conclude that the recent decline in vacancies appears consistent with tighter monetary policy because industries that had higher exposure to monetary policy tightening have seen larger declines in vacancies since the federal funds rate started increasing in early 2022. This finding suggests that monetary policy tightening appears, at least in part, responsible for the decline in vacancies from March 2022 through December 2022.
Have Vacancies Declined Further in Locations in Which Monetary Policy Tightening Rises Financing Costs Further?
For our second exercise, we look for variation across states in the effects of monetary policy tightening on financing costs. Ideally, we would look to measure variation across states in how financing costs increase when monetary policy tightens; however, to our knowledge there are no direct data measuring this. In this exercise, then, we combine aspects of two different kinds of literature to construct a proxy for our ideal measure.
Literature documents differences in interest rate spreads—the interest rate banks charge on loans to private-sector customers minus that paid by commercial or similar banks—across countries. For instance, Carey and Nini (2007) present evidence of differences in spreads at the corporate level between the United States and European countries and how that difference cannot be explained by borrower, loan, and lender characteristics. They attribute part of these differences to home bias, but they also acknowledge that the main reasons remain a puzzle. Moreover, they present evidence that the difference in spreads increases when the cost of financing increases.6
Second, there is literature that shows that, in the United States, there are significant differences in mortgage rates for identical borrower and loan profiles across metropolitan statistical areas (MSAs). For instance, Hurst et al. (2016) show that there is a significant difference in privately securitized mortgage rates at the MSA level, one that cannot be explained by the characteristics of the borrower or the loan.7 However, they do not present any evidence on whether mortgage rates, after accounting for loan and mortgage characteristics, change differently across states when mortgage rates increase overall.
Our analysis combines both ideas to create a proxy for our ideal measure. We estimate how much mortgage rates increase for each state when monetary policy tightens, and we use the small differences across states in these increases as our proxy for how business financing costs would increase in these same states when the federal funds rate increases. To construct it we use the following strategy.
Using microdata on individual mortgage loans from the 2021 HMDA data, we use cross-state variation to estimate how much mortgage rates, for an identical loan and borrower profile, change across states when the average prime offer rate (APOR) changes8 Since we do not see time variation in the federal funds rate in our data, here we make our first assumption. We assume that changes in the APOR (for which we see some small time-variation in our data) are equivalent to changes in the federal funds rate. We base this assumption on the behavior of the APOR and the federal funds rate over the last few years. As shown in Figure B.1 in the appendix, the APOR for 15-year and 30-year mortgages co-moves with the federal funds rate even if their changes are not perfectly aligned and the APOR shows more volatility.
Our empirical strategy uses a linear regression to estimate the effect of changes in the APOR on mortgage rates in each state after controlling for a vast set of loan, borrower, and property characteristics. We denote our coefficients of interest as γs. Each γs coefficient represents the increase in mortgage rates in state s relative to the increase in our baseline state for identical loan and borrower characteristics when the APOR increases by 100 basis points. If we additionally use our first assumption, that the changes in the APOR are equivalent to those in the federal funds rate, our estimated γs coefficients will not only represent how mortgage rates for identical loan and borrower characteristics differentially change across states when the APOR increases, but they will also capture the differential effect of changes in the federal funds rate on mortgage rates across states.
We find that, while small,9 there is significant variation across states regarding how much mortgage rates increase for identical borrower and loan characteristics when the APOR increases.10 Why would mortgage rates increase differently across states when the APOR increases? Previous work provides some suggestions as to why this might be the case. For instance, Bhutta et al. (2020) explain how lender rate sheets depend not only on borrower and loan characteristics, but also on geographic location. Depending on the structure of these adjustments, it is possible that the effect of these adjustments on the final mortgage rate varies depending on the level of the APOR. A different explanation comes from Altavilla et al. (2020), who document that in the eurozone, pass-through from monetary policy to lending rates depended on a bank’s characteristics such as its balance sheet characteristics, level of capitalization, exposure to sovereign debt, and share of nonperforming loans. If US states each have different compositions of lenders with different characteristics, we could expect the effects of increases in rates to result in different increases in mortgage rates across states, even if the effects are not location specific, per se.
Using the estimated γs coefficients, one for each state, as our proxy for the ideal measure requires us to make a critical second assumption, namely, that the estimated coefficients not only represent how mortgage rates change across states when the federal funds rate rises, but also that they represent how business financing costs change across states when the federal funds rate increases. This assumption is reasonable if the reason why borrowing costs increase differentially across states when monetary policy tightens comes from the different composition of lenders in each state, a circumstance which should affect the passthrough from monetary policy to borrowing rates, not only for mortgages, but also for other types of loans. Similarly, if the differences in the change in mortgage rates across states when monetary policy tightens arise from the differences in state-specific regulations that affect both the mortgage and business credit markets, this assumption appears also likely to hold. Under this assumption, the estimated γs coefficients provide the required cross-state variation capturing how much business financing costs increase across states for identical types of loans and borrowers when monetary policy tightens.
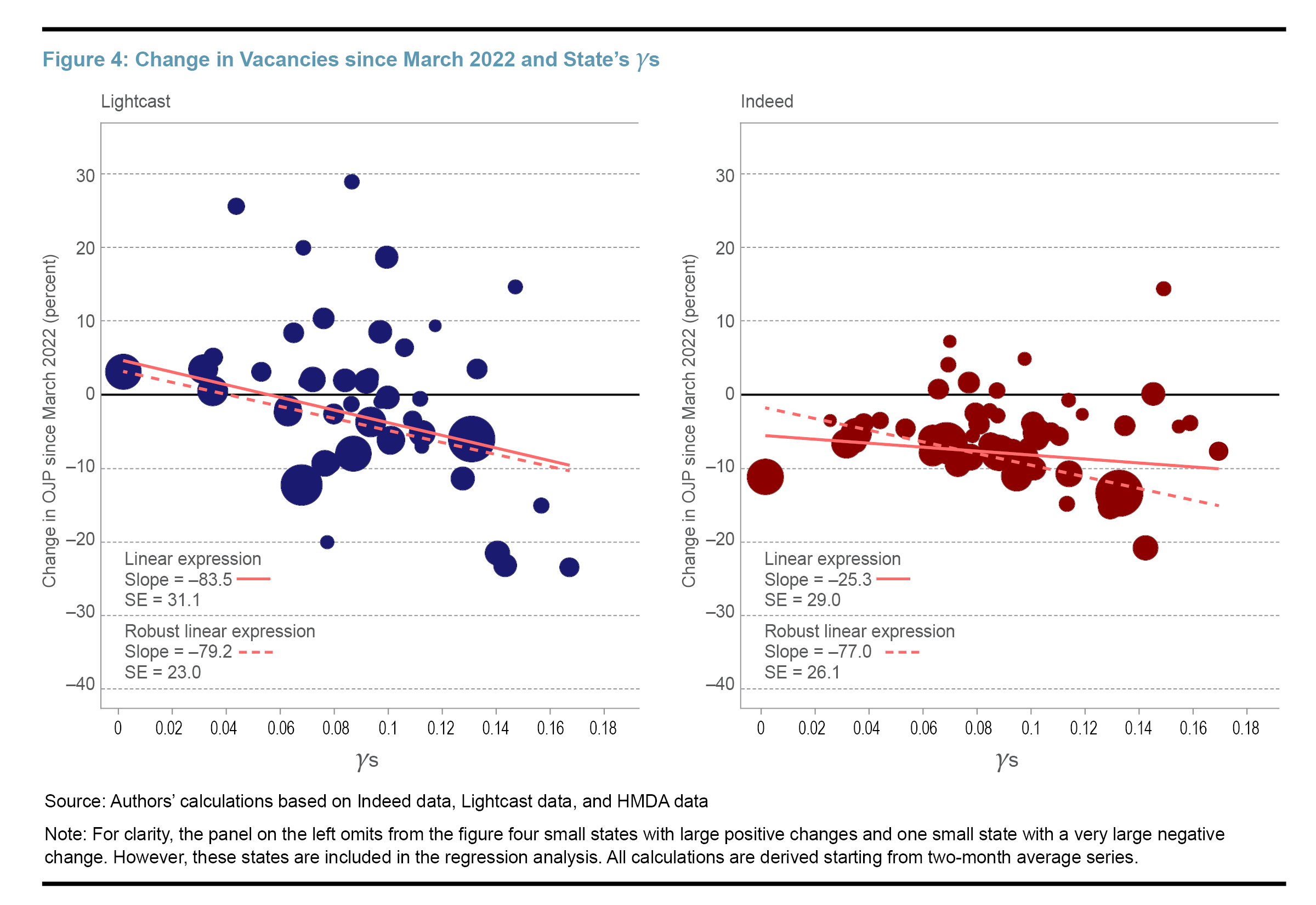
Armed with our estimated γs coefficients, we now test whether states in which increases in the federal funds rate translate into larger increases in business financing costs have seen larger declines in vacancies since March 2022. The results are shown in Figure 4. The x-axis shows the estimated γs coefficients, which are normalized so that the state with the lowest value takes the value 0 and all other states are expressed relative to it. The y-axis shows the change in online job postings at the state level from two different sources of data: Lightcast (left) and Indeed (right).11,12 The solid lines in Figure 4 show the estimated relationship between the change in online job postings since March 2022 and the estimated γs coefficients at the state level. For both Lightcast and Indeed, the decline in online job postings has been larger in states in which tighter financial conditions result in relatively higher financing costs, as evidenced by the negative slope estimated in both panels. We additionally test whether outliers could be behind the estimated results. As shown in the dashed lines of Figure 4, accounting for outliers as described in Jann (2022) does not modify our results and, in fact, strengthens our conclusions.
Conclusion
The focus of this Economic Commentary is to highlight two different approaches to assess the effects of monetary policy on the labor market. Our first focuses on variation at the industry level in exposure to tighter financial conditions. Our second exploits geographic differences in the effect of monetary policy tightening on financing costs. The results for both suggest that monetary policy tightening is already having a measurable impact on the labor market through the vacancy creation channel.
Leveraging cross-sectional variation across industries and states allows us to provide more direct and stronger evidence of the effects of monetary policy tightening on the labor market when compared to simply considering the evolution of vacancies at the national level since the FOMC lifted the federal funds rate. In the case of the latter analysis, it is difficult to argue that the changes are driven by monetary policy and do not arise from the natural evolution of the labor market or some other dimension. By exploiting the differential effects on the tightening of financial conditions in the cross-section of national monetary policy tightening, however, we circumvent the previous difficulties. For our results to be driven by something different than monetary policy tightening, we would require that the unobserved dimension is correlated with the differential tightening of financial conditions across states and industries, a situation which seems significantly less likely.
The limitations of the available data and the strong assumptions we impose in our empirical analysis make us cautious of putting too much weight on the estimated parameters in our regressions other than assessing its sign. Thus, we argue that monetary policy had an impact on vacancy creation, but we refrain from estimating by how much or by how much more tightening would have to increase in order to bring labor supply and demand back in line. Further research along these dimensions would be extremely useful.
Endnotes
- The data used for the different series is the latest available data as of mid-February 2023. Return to 1
- We use March 2022 as our starting period because that is when the federal funds rate was first raised in the pandemic period. One could argue that monetary policy started tightening even before the federal funds rate was raised. For instance, on January 26, 2022, the FOMC declared that it expected it would soon be appropriate to raise the target range for the federal funds rate. We test the sensitivity of the results shown in this Economic Commentary to the choice of the starting date. We find very little difference in our results regardless of whether we set this date in February or March 2022. Return to 2
- See https://www.census.gov/econ/qfr/index.html. Return to 3
- The QFR defines current liabilities as “short-term debt with an original maturity of 1 year or less and installments, due in 1 year or less, on long-term debt.” The QFR defines current assets as “total cash, US government and other securities, inventories and trade accounts, and trade note receivables.” Return to 4
- In each regression, each industry is weighted using the industry’s employment in March 2022 for JOLTS. Return to 5
- In their results, the difference between spreads in the United States and Europe increased by 10 to 20 basis points after 1999, a period in which the cost of financing increased, coincident with increased corporate bond default rates that moved spreads, in general, higher. Return to 6
- Hurst et al. (2016) document that differences in privately securitized mortgage rates across MSAs are correlated with predictable local default risk. Return to 7
- HMDA defines the average prime offer rate for mortgages as the hypothetical mortgage rate offered to a “best quality,” 80 percent loan-to-value, first-lien loan for a given loan duration. The APOR does not vary at the state level. Beyond the loan’s interest rate, each loan in the HMDA dataset has an average prime offer rate attached to it, from which it is possible to calculate the interest rate spread of the specific loan. Return to 8
- The standard deviation of the estimated γs is just 3.6 basis points. Table B.1 in the appendix shows the estimated coefficients for each state. Return to 9
- To test whether the estimated γs coefficients capture an economically meaningful magnitude, we examine their correlation with the change in home sales, at the state level, from March to December 2022. Since during this period “rates” increased significantly, we would expect states with larger coefficients (that is, states where an increase in the APOR results in a larger increase in mortgage rates for identical borrower and loan characteristics) to have seen significantly larger declines in home sales. We find that to be the case, as shown in Figure A.1 in the appendix. We see this as a clear indication that the estimates capture an economically meaningful variation whose effects translate into meaningful changes in home sales across states. Return to 10
- The Bureau of Labor Statistics (BLS) provides model-based JOLTS estimates of job openings at the state level. However, we do not use them for this exercise. JOLTS is designed to provide a national estimate of job openings, but, as we discuss in detail in the appendix, its sample size is potentially too small to provide precise estimates of recent changes in job openings at the state level, especially if those changes vary significantly across states within the same census region. Return to 11
- The y-axis on the figure on the left-hand side shows the change in open job postings (OJP) from Lightcast from March 2022 until December 2022 (latest available data). On the right-hand side panel, the y-axis shows the change in OJP from Indeed from March 2022 until January 2023 (latest available data). Return to 12
References
- Altavilla, Carlo, Fabio Canova, and Matteo Ciccarelli. 2020. “Mending the Broken Link: Heterogeneous Bank Lending Rates and Monetary Policy Pass-Through.” Journal of Monetary Economics 110 (April): 81–98. https://doi.org/10.1016/j.jmoneco.2019.01.001.
- Bhutta, Neil, Andreas Fuster, and Aurel Hizmo. 2020. “Paying Too Much? Price Dispersion in the US Mortgage Market.” Finance and Economics Discussion Series 2020–062. Board of Governors of the Federal Reserve System. https://doi.org/10.17016/FEDS.2020.062.
- Brunow, Stephan, Stefanie Lösch, and Ostap Okhrin. 2022. “Labor Market Tightness and Individual Wage Growth: Evidence from Germany.” Journal for Labour Market Research 56 (1): 16. https://doi.org/10.1186/s12651-022-00322-7.
- Cantor, Richard. 1990. “Effects of Leverage on Corporate Investment and Hiring Decisions.” Quarterly Review 15 (Sum): 31–41. https://ideas.repec.org//a/fip/fednqr/y1990isump31-41nv.15no.2.html.
- Carey, Mark, and Greg Nini. 2007. “Is the Corporate Loan Market Globally Integrated? A Pricing Puzzle.” The Journal of Finance 62 (6): 2969–3007. https://doi.org/10.1111/j.1540-6261.2007.01298.x.
- Domash, Alex, and Lawrence H. Summers. 2022. “How Tight Are US Labor Markets?” Working Paper Series 29739. National Bureau of Economic Research. https://doi.org/10.3386/w29739.
- Garibaldi, Pietro. 1997. “The Asymmetric Effects of Monetary Policy on Job Creation and Destruction.” IMF Staff Papers 44 (4). https://www.elibrary.imf.org/view/journals/024/1997/004/article-A007-en.xml.
- Ghosh, Saibal, and Saurabh Ghosh. 2006. “Does Monetary Policy Affect a Firm’s Investment Through Leverage? Micro Evidence for India.” Economia Internazionale / International Economics 59 (1): 17–31. https://ideas.repec.org//a/ris/ecoint/0089.html.
- Hu, Charles X. 1999. “Leverage, Monetary Policy, and Firm Investment.” Economic Review 1999 (2): 32–39. https://ideas.repec.org//a/fip/fedfer/y1999p32-39n2.html.
- Hurst, Erik, Benjamin J. Keys, Amit Seru, and Joseph Vavra. 2016. “Regional Redistribution through the US Mortgage Market.” American Economic Review 106 (10): 2982–3028. https://doi.org/10.1257/aer.20151052.
- Jann, Ben. 2022. “ROBREG: Stata Module Providing Robust Regression Estimators.” Statistical Software Components. https://econpapers.repec.org/software/bocbocode/S458931.htm.
- Jordà, Òscar, Celeste Liu, Fernanda Nechio, and Fabián Rivera-Reyes. 2022. “Wage Growth When Inflation Is High.” FRBSF Economic Letter 2022 (25): 1–6. https://ideas.repec.org//a/fip/fedfel/94744.html.
- Petrosky-Nadeau, Nicolas. 2014. “Credit, Vacancies and Unemployment Fluctuations.” Review of Economic Dynamics 17 (2): 191–205. https://doi.org/10.1016/j.red.2013.10.001.
- Powell, Jerome H. 2022. “Inflation and the Labor Market: Speech at the Hutchins Center on Fiscal and Monetary Policy, Brookings Institution, Washington DC. November 30, 2022.” Board of Governors of the Federal Reserve System. https://fedinprint.org/item/fedgsq/95821.
Suggested Citation
Bailey, Adiah, and Victor Hernandez Martinez. 2023. “The Effect of Higher Financing Costs on Job Openings and Online Job Postings.” Federal Reserve Bank of Cleveland, Economic Commentary 2023-09. https://doi.org/10.26509/frbc-ec-202309
This work by Federal Reserve Bank of Cleveland is licensed under Creative Commons Attribution-NonCommercial 4.0 International
- Share