How Successful Is Your Region at Retaining Domestic Migrants?
For regions in the Fourth District and across the United States, this District Data Brief analyzes how well each region retains domestic migrants, or those who move in from other parts of the country. It also addresses the extent to which retention rates are associated with population growth.
The views authors express in District Data Briefs are theirs and not necessarily those of the Federal Reserve Bank of Cleveland or the Board of Governors of the Federal Reserve System. The series editor is Harrison Markel.
Introduction
As the US population ages and the natural increase (defined as births minus deaths) declines in more regions, attracting residents from other parts of the country, or “domestic migrants,” is becoming increasingly important for maintaining a given region’s population and workforce. Local leaders often believe that once someone has lived in their area, this person will want to stay. How often is this true? In this District Data Brief, we use the long histories of individuals’ locations drawn from the Federal Reserve Bank of New York/Equifax Consumer Credit Panel to measure the retention of migrants who have moved to regions across the country. This analysis builds on our recent District Data Brief, “How Successful Is Your Region at Retaining Its Native Residents?” We find that metro areas with faster growth rates retain a higher percentage of their in-migrants and that population growth is highest if a region combines high retention with robust in-migration. In the Fourth Federal Reserve District,1 only Columbus and Lexington successfully translate high retention of migrants into population growth.
Data and Definitions
The estimates of individuals’ long-run patterns of migration are created with a random, anonymous sample drawn from credit histories maintained by Equifax, known as the Federal Reserve Bank of New York/Equifax Consumer Credit Panel (CCP). Almost nine of 10 adults in the United States have accounts with creditors (for example, mortgages, student loans, auto loans, and credit cards), and these lenders report billing addresses to the credit bureaus each month. The CCP data include the county that contains the borrower’s billing address, and this enables us to observe each quarter whether an individual is living in their home region or another region. When borrowers first apply for credit, we designate them as a native of the region in which they are living.2 Because the CCP begins in 1999, we must limit the analysis to people born in 1981 or later, as credit histories do not start until age 18 (typically between 18 and 23), and we need to observe people when their credit history begins to accurately place them in their home region.
Some of the results presented below are disaggregated by credit score. The score available in the CCP is the Equifax Risk Score. Like other credit scores, it uses information in borrowers’ credit records to predict the probability of their becoming delinquent on debts.
In this District Data Brief, the term “metro” refers to a Core-Based Statistical Area (CBSA) as defined by the Office of Management and Budget (OMB). The US Department of Agriculture groups rural counties into regions called “commuting zones” (CZs) based on how frequently people drive between the counties for work. We use the CZ definitions for all nonmetro counties so that we can include all counties in our calculations. We define “large metros” as those with populations of greater than 1 million for graphs of the top-10 and bottom-10 metros. To better illustrate certain relationships, the samples in scatterplots include all regions with populations of greater than 500,000.
Retention Estimates
To measure domestic migrant retention, we begin by identifying every person represented in the CCP data who moves away from their home region. For each destination region to which they move, we look at all the quarters we can observe for the arriving migrants, from the time of their first arrival through the second quarter of 2024, the most recent quarter for which data are available. We then calculate the share of those observed quarters during which the migrants were reported to still be living in that destination. We refer to this ratio as the migrant retention rate; it tells us how successful the region is at retaining people who have arrived from elsewhere.
The top 10 metros in the nation as ranked by migrant retention rate are almost all among the fastest growing in the country. Among them, Denver, Nashville, and Austin have seen particularly strong growth over the last two decades. By contrast, the bottom 10 metros as ranked by migrant retention include mostly slow-growing or shrinking places including Buffalo, Rochester, and Providence. One Fourth District metro, Cleveland, is among the bottom 10.
In Figure 1, the green bars represent the migrant retention measure calculated for individuals with Equifax Risk Scores in the top third of the distribution. Higher scores indicate these borrowers have consistently paid their debts on time, suggesting they probably have in-demand skills and strong earning power. In some of the more expensive metros, including Denver, Seattle, San Diego, and Honolulu, in-migrants with higher Equifax Risk Scores are more likely to stay than in-migrants with lower scores.
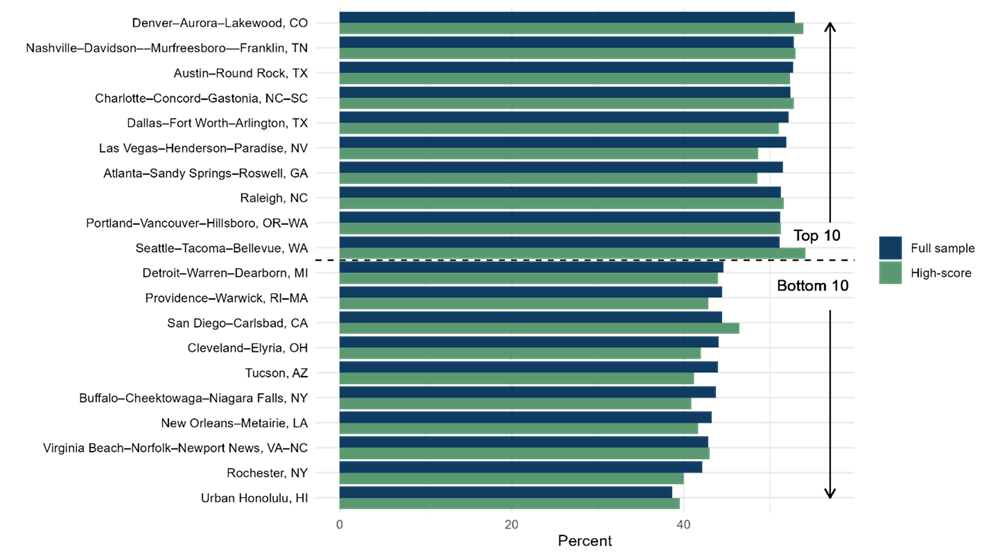
Sources: Federal Reserve Bank of New York/Equifax Consumer Credit Panel and authors’ calculations. “High-score” estimates limit the sample to individuals with Equifax Risk Scores in the top third of the distribution.
In Figure 2, Fourth District metros’ migrant retention rates are graphed alongside the average for all metros and CZs. Columbus and Lexington are the only two District metros that retain their migrants at an above-average rate. Our District Data Brief last month reported that native retention is higher in larger metro areas, and we now find that this holds true for domestic migrant retention as well. Metros with populations of above 1 million have an average migrant retention rate of 48.5 percent, while those with populations of below 1 million have an average rate of 42.3 percent.3 This suggests that scale provides an advantage in retaining residents.
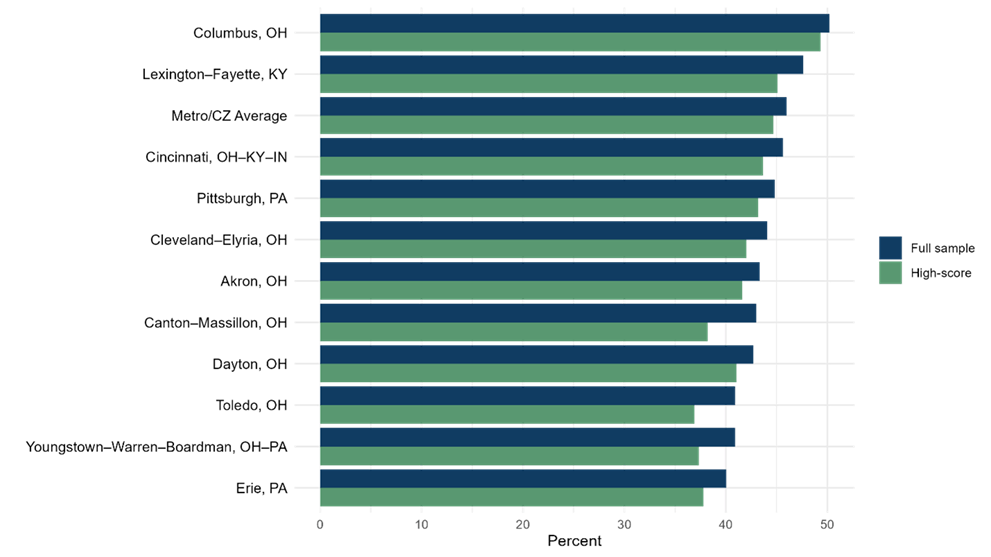
Sources: Federal Reserve Bank of New York/Equifax Consumer Credit Panel and authors’ calculations. “High-score” estimates limit the sample to individuals with Equifax Risk Scores in the top third of the distribution.
Figure 3 plots regions’ migrant retention against their average annual population change from 2000 to 2020. The vertical and horizontal lines represent the averages of their respective measures, while the diagonal line is a population-weighted line of best fit. Overall, metros with higher average migrant retention also have faster-growing populations. This relationship is visible among the Fourth District metros (labeled in green) and among all regions with over 500,000 residents (indicated with blue markers).
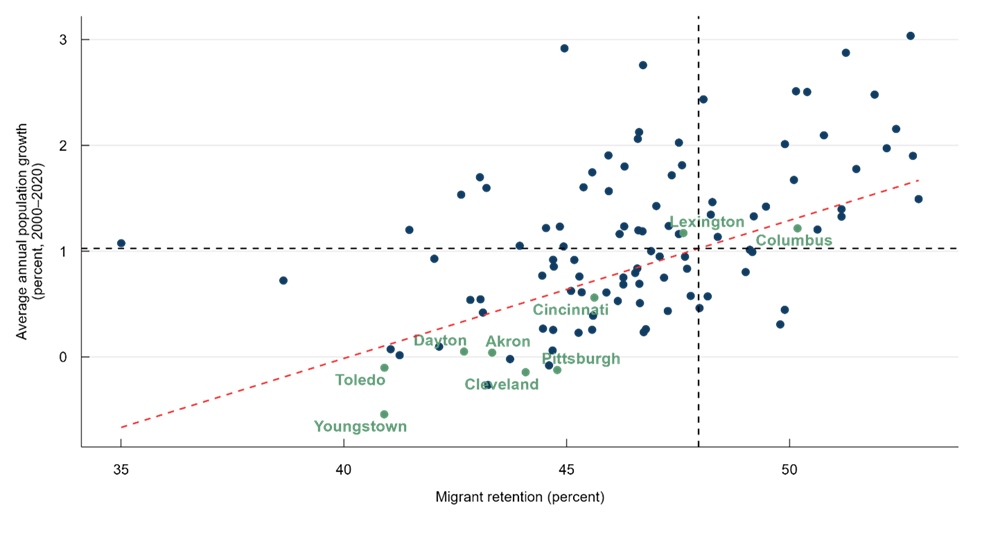
Sources: Census Bureau, Federal Reserve Bank of New York/Equifax Consumer Credit Panel, and authors’ calculations. Fourth District metro areas are shown with green markers and abbreviated labels.
At any given retention rate, the more migrants that arrive, the more they can contribute to the population. So, which of these measures matters most: native retention, migrant inflow, or migrant retention? To figure this out, we can sort all regions into categories based on whether they score above- or below-average on each measure. These categories and their average population growth rates are shown in Table 1.
For Akron, Canton, Dayton, Erie, Toledo, and Youngstown, raising any of these measures above the national average would probably help increase their population growth. For Cincinnati, Cleveland, and Pittsburgh, this simple breakdown suggests focusing on increasing migrant retention because the regions with high native retention, low inflows, and high migrant retention fared better (8.2 percent population growth) than regions with high native retention, high inflows, and low migrant retention (5.1 percent).
Table 1. Population Growth by Level of Retention and Migrant Inflows
Native retention | Migrant inflow | Migrant retention | Average population growth (2000–2020) | Most populous metro in category | Fourth District metros in category |
Low | High | High | 17.2 | Orlando | Lexington |
High | High | High | 15.1 | Washington | Columbus |
High | Low | High | 8.2 | New York | |
Low | High | Low | 5.7 | Virginia Beach | |
High | High | Low | 5.1 | San Diego | |
Low | Low | High | 3.9 | Allentown | |
High | Low | Low | 2.5 | Detroit | Cincinnati, Cleveland, Pittsburgh |
Low | Low | Low | –0.3 | Bridgeport, CT | Akron, Canton, Dayton, Erie, Toledo, Youngstown |
Note: “High” indicates regions with above-average values of the measure, and “Low” indicates below-average values of the measure.
Sources: US Census Bureau, Federal Reserve Bank of New York/Equifax Consumer Credit Panel, and author’s calculations.
Conclusion
Our analysis shows that regions can expect to keep migrants for about 40 to 50 percent of the migrants’ time after they arrive. Domestic migration, although undergoing a steady decline, has become the main source of population expansion for growing regions. We have shown here that, in most cases, metros that are better at retaining domestic migrants have faster-growing populations.
Retaining domestic migrants translated into strong population growth for Lexington and Columbus because they also have substantial inflows of domestic migrants. With these domestic migrant retention measures in hand, the Fourth District regions with weaker population growth can explore whether there are effective ways to emulate the regions with higher migrant retention rates and whether these would be worth the necessary investments.
Footnotes
- The Fourth District covers Ohio, western Pennsylvania, eastern Kentucky, and the northern panhandle of West Virginia. Return to 1
- Some students might use a college dormitory address in their first application for credit. If they are attending school out of town, they may be labeled natives of the wrong region. This could bias the estimates of retention, especially for college towns, because these students are likely to return home or move on after graduation. To account for this, we estimate the relationship between regions’ student populations and their retention. We then adjust each region’s retention estimate to remove the effect of students. Return to 2
- These are population-weighted averages of the retention rates of the regions, CBSAs, or CZs. Without population weighting, the averages are 47.9 percent for regions with populations of above 1 million and 40.1 percent for regions with populations of under 1 million. The overall population-weighted national average is 45.8 percent, and the unweighted average is 40.3 percent. Return to 3
Appendix
Table A1. Share of Migrants’ Quarters Spent in Their Destination Metro (Population >500,000)
Metro area | Migrant retention rate | Metro area | Migrant retention rate | ||
Full sample | High-score | Full sample | High-score | ||
Denver–Aurora–Lakewood, CO | 52.90 | 53.92 | Tulsa, OK | 46.58 | 44.27 |
Nashville–Davidson–Murfreesboro–Franklin, TN | 52.77 | 52.99 | Lancaster, PA | 46.54 | 44.88 |
Austin–Round Rock, TX | 52.72 | 52.34 | Ogden–Clearfield, UT | 46.30 | 46.81 |
Charlotte–Concord–Gastonia, NC–SC | 52.39 | 52.79 | Columbia, SC | 46.29 | 42.81 |
Dallas–Fort Worth–Arlington, TX | 52.18 | 51.04 | Allentown–Bethlehem–Easton, PA–NJ | 46.27 | 42.69 |
Las Vegas–Henderson–Paradise, NV | 51.91 | 48.63 | San Francisco–Oakland–Hayward, CA | 46.27 | 49.67 |
Atlanta–Sandy Springs–Roswell, GA | 51.50 | 48.54 | Fresno, CA | 46.19 | 43.52 |
Raleigh, NC | 51.26 | 51.64 | Baltimore–Columbia–Towson, MD | 46.15 | 45.95 |
Portland–Vancouver–Hillsboro, OR–WA | 51.17 | 51.27 | Bakersfield, CA | 45.94 | 43.23 |
Seattle–Tacoma–Bellevue, WA | 51.17 | 54.13 | Charleston–North Charleston, SC | 45.94 | 44.50 |
Houston–The Woodlands–Sugar Land, TX | 50.77 | 49.34 | Wichita, KS | 45.89 | 43.06 |
Indianapolis–Carmel–Anderson, IN | 50.63 | 49.95 | Cincinnati, OH–KY–IN | 45.62 | 43.66 |
Fayetteville–Springdale–Rogers, AR–MO | 50.40 | 49.54 | Bridgeport–Stamford–Norwalk, CT | 45.59 | 45.11 |
Columbus, OH | 50.18 | 49.32 | North Port–Sarasota–Bradenton, FL | 45.57 | 43.10 |
Boise City, ID | 50.14 | 50.00 | Hartford–West Hartford–East Hartford, CT | 45.57 | 44.36 |
Des Moines–West Des Moines, IA | 50.10 | 51.22 | Stockton–Lodi, CA | 45.38 | 43.52 |
Phoenix–Mesa–Scottsdale, AZ | 49.90 | 48.55 | Portland–South Portland, ME | 45.34 | 45.42 |
New York–Newark–Jersey City, NY–NJ–PA | 49.89 | 50.44 | Harrisburg–Carlisle, PA | 45.28 | 43.08 |
Los Angeles–Long Beach–Anaheim, CA | 49.79 | 50.18 | New Haven–Milford, CT | 45.27 | 41.38 |
Tampa–St. Petersburg–Clearwater, FL | 49.47 | 48.13 | Baton Rouge, LA | 45.17 | 41.56 |
Oklahoma City, OK | 49.20 | 47.21 | Worcester, MA–CT | 45.10 | 43.57 |
Minneapolis–St. Paul–Bloomington, MN–WI | 49.17 | 49.76 | Provo–Orem, UT | 44.95 | 44.00 |
Little Rock–North Little Rock–Conway, AR | 49.11 | 47.28 | Modesto, CA | 44.93 | 43.38 |
Louisville/Jefferson County, KY–IN | 49.01 | 46.79 | Spokane–Spokane Valley, WA | 44.85 | 42.45 |
Albuquerque, NM | 48.39 | 46.78 | Pittsburgh, PA | 44.79 | 43.17 |
Salt Lake City, UT | 48.27 | 48.21 | Winston–Salem, NC | 44.71 | 42.59 |
Washington–Arlington–Alexandria, DC–VA–MD–WV | 48.23 | 49.87 | St. Louis, MO–IL | 44.70 | 42.73 |
Boston–Cambridge–Newton, MA–NH | 48.16 | 48.46 | Greensboro–High Point, NC | 44.69 | 40.62 |
Orlando–Kissimmee–Sanford, FL | 48.07 | 47.03 | Scranton–Wilkes–Barre–Hazleton, PA | 44.68 | 39.21 |
Philadelphia–Camden–Wilmington, PA–NJ–DE–MD | 47.98 | 46.55 | Detroit–Warren–Dearborn, MI | 44.60 | 43.98 |
Birmingham–Hoover, AL | 47.78 | 46.45 | Palm Bay–Melbourne–Titusville, FL | 44.54 | 44.41 |
Chattanooga, TN–GA | 47.70 | 46.50 | Providence–Warwick, RI–MA | 44.47 | 42.85 |
Kansas City, MO–KS | 47.65 | 47.73 | San Diego–Carlsbad, CA | 44.45 | 46.46 |
Lexington–Fayette, KY | 47.62 | 45.08 | Cleveland–Elyria, OH | 44.08 | 42.00 |
Jacksonville, FL | 47.59 | 47.35 | Tucson, AZ | 43.95 | 41.17 |
San Antonio–New Braunfels, TX | 47.52 | 45.60 | Buffalo–Cheektowaga–Niagara Falls, NY | 43.73 | 40.86 |
Omaha–Council Bluffs, NE–IA | 47.52 | 47.00 | Akron, OH | 43.33 | 41.60 |
Riverside–San Bernardino–Ontario, CA | 47.36 | 46.42 | New Orleans–Metairie, LA | 43.23 | 41.66 |
Greenville–Anderson–Mauldin, SC | 47.29 | 43.85 | Durham–Chapel Hill, NC | 43.20 | 41.44 |
Jackson, MS | 47.27 | 43.80 | Albany–Schenectady–Troy, NY | 43.12 | 40.61 |
Grand Rapids–Wyoming, MI | 47.18 | 47.23 | Oxnard–Thousand Oaks–Ventura, CA | 43.07 | 43.64 |
Knoxville, TN | 47.09 | 44.06 | Colorado Springs, CO | 43.05 | 41.34 |
Sacramento–Roseville–Arden–Arcade, CA | 47.01 | 45.54 | Virginia Beach–Norfolk–Newport News, VA–NC | 42.84 | 43.00 |
Miami–Fort Lauderdale–West Palm Beach, FL | 46.89 | 44.72 | Dayton, OH | 42.70 | 41.03 |
Chicago–Naperville–Elgin, IL–IN–WI | 46.78 | 46.96 | Deltona–Daytona Beach–Ormond Beach, FL | 42.63 | 38.21 |
Milwaukee–Waukesha–West Allis, WI | 46.73 | 45.75 | Rochester, NY | 42.14 | 39.97 |
Cape Coral–Fort Myers, FL | 46.71 | 43.85 | Augusta–Richmond County, GA–SC | 42.03 | 39.86 |
Richmond, VA | 46.70 | 46.23 | El Paso, TX | 41.47 | 38.51 |
Memphis, TN–MS–AR | 46.64 | 42.72 | Springfield, MA | 41.25 | 37.01 |
San Jose–Sunnyvale–Santa Clara, CA | 46.63 | 48.13 | Syracuse, NY | 41.05 | 38.26 |
McAllen–Edinburg–Mission, TX | 46.62 | 40.90 | Toledo, OH | 40.91 | 36.90 |
Madison, WI | 46.61 | 46.53 | Youngstown–Warren–Boardman, OH–PA | 40.91 | 37.34 |
Lakeland–Winter Haven, FL | 46.60 | 42.53 | Urban Honolulu, HI | 38.64 | 39.54 |
Sources: Federal Reserve Bank of New York/Equifax Consumer Credit Panel and authors' calculations. “High-score” estimates limit the sample to individuals with Equifax Risk Scores in the top third of the distribution.
Table A2. Share of Migrants’ Quarters Spent in Their Destination Metro (Population-Weighted Average, Population ≤500,000)
Area | Migrant retention rate | Area | Migrant retention rate | ||
Full sample | High-score | Full sample | High-score | ||
Small metro and rural – Nevada | 45.17 | 44.52 | Small metro and rural – Oregon | 41.05 | 38.65 |
Small metro and rural – New Jersey | 43.59 | 42.27 | Small metro and rural – Virginia | 41.03 | 37.87 |
Small metro and rural – Maryland | 43.43 | 41.98 | Small metro and rural – Colorado | 40.94 | 39.80 |
Small metro and rural – Alabama | 43.42 | 39.76 | Small metro and rural – Washington | 40.84 | 39.67 |
Small metro and rural – Louisiana | 43.14 | 39.01 | Small metro and rural – Illinois | 40.81 | 37.41 |
Small metro and rural – Tennessee | 43.09 | 40.26 | Small metro and rural – Montana | 40.79 | 39.20 |
Small metro and rural – South Carolina | 42.97 | 40.43 | Small metro and rural – Florida | 40.78 | 37.49 |
Small metro and rural – Delaware | 42.91 | 41.60 | Small metro and rural – Missouri | 40.75 | 36.97 |
Small metro and rural – Vermont | 42.89 | 41.63 | Small metro and rural – Ohio | 40.72 | 37.33 |
Small metro and rural – New Hampshire | 42.68 | 42.11 | Small metro and rural – California | 40.71 | 39.09 |
Small metro and rural – Hawaii | 42.34 | 40.88 | Small metro and rural – Michigan | 40.56 | 38.67 |
Small metro and rural – Arkansas | 42.33 | 36.63 | Small metro and rural – New Mexico | 40.43 | 35.83 |
Small metro and rural – Kentucky | 42.30 | 38.37 | Small metro and rural – Idaho | 40.40 | 39.14 |
Small metro and rural – Alaska | 42.16 | 43.68 | Small metro and rural – Utah | 40.36 | 39.51 |
Small metro and rural – West Virginia | 42.06 | 36.95 | Small metro and rural – Texas | 40.34 | 35.43 |
Small metro and rural – Maine | 41.94 | 38.99 | Small metro and rural – Massachusetts | 40.18 | 37.44 |
Small metro and rural – Pennsylvania | 41.93 | 39.84 | Small metro and rural – South Dakota | 40.16 | 38.13 |
Small metro and rural – North Carolina | 41.82 | 39.18 | Small metro and rural – Oklahoma | 40.04 | 34.58 |
Small metro and rural – Georgia | 41.63 | 38.14 | Small metro and rural – New York | 39.84 | 37.50 |
Small metro and rural – Mississippi | 41.38 | 36.83 | Small metro and rural – Nebraska | 39.33 | 36.98 |
Small metro and rural – Indiana | 41.20 | 38.29 | Small metro and rural – Iowa | 38.63 | 36.16 |
Small metro and rural – Wyoming | 41.17 | 41.35 | Small metro and rural – Arizona | 38.32 | 35.49 |
Small metro and rural – North Dakota | 41.12 | 39.02 | Small metro and rural – Minnesota | 38.26 | 36.73 |
Small metro and rural – Connecticut | 41.10 | 40.29 | Small metro and rural – Kansas | 37.80 | 34.75 |
Small metro and rural – Wisconsin | 41.09 | 39.35 |
Sources: Federal Reserve Bank of New York/Equifax Consumer Credit Panel and authors’ calculations. “High-score” estimates limit the sample to individuals with Equifax Risk Scores in the top third of the distribution.
Suggested Citation
Whitaker, Stephan D., and Brett Huettner. 2024. “How Successful Is Your Region at Retaining Domestic Migrants?” Federal Reserve Bank of Cleveland, Cleveland Fed District Data Brief. https://doi.org/10.26509/frbc-ddb-20241121
This work by Federal Reserve Bank of Cleveland is licensed under Creative Commons Attribution-NonCommercial 4.0 International
- Share